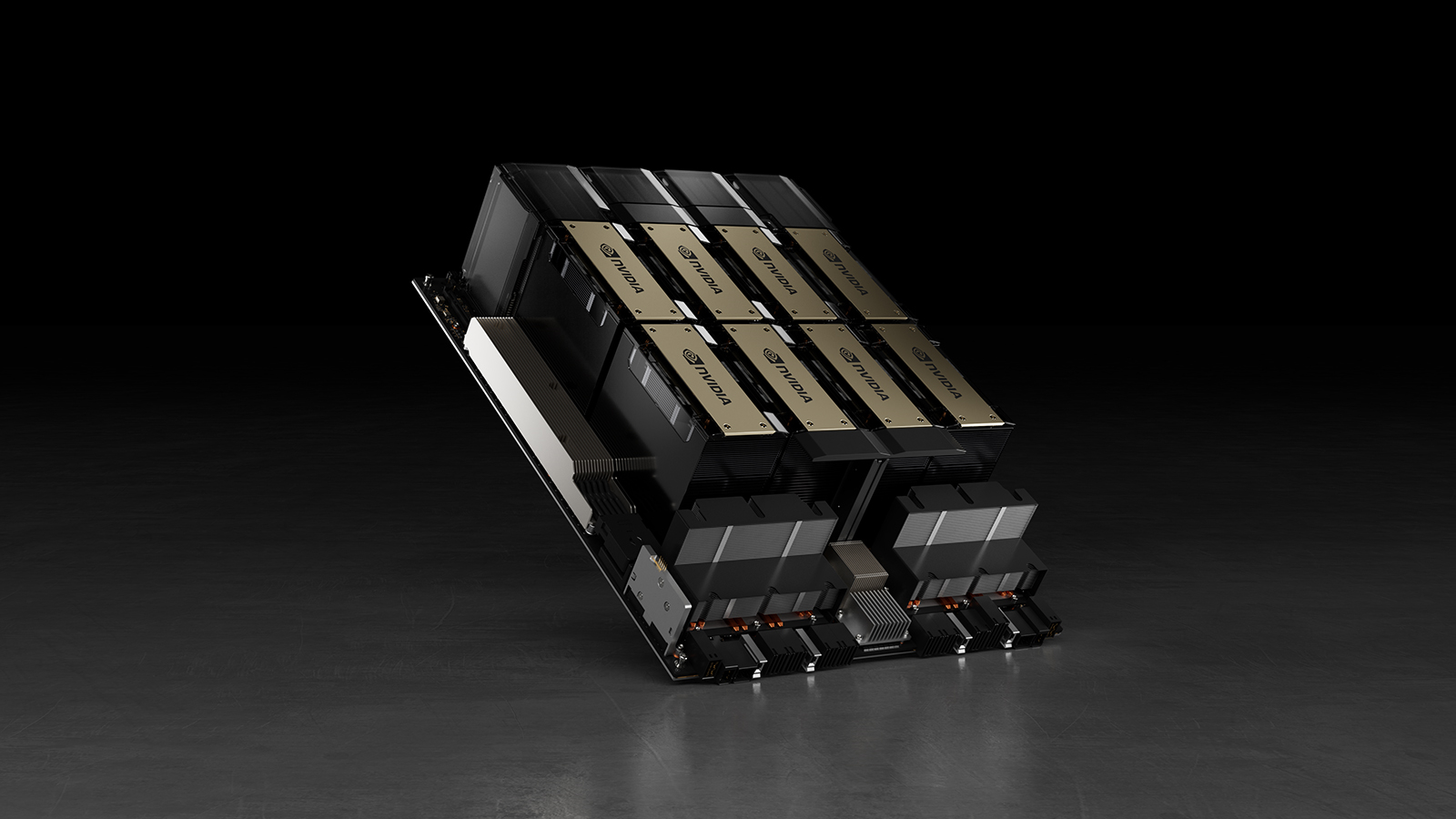
At its Instinct MI300X launch AMD asserted that its latest GPU for artificial intelligence (AI) and high-performance computing (HPC) is significantly faster than Nvidia's H100 GPU in inference workloads. Nvidia this week took time to show that the situation is quite the opposite: when properly optimized, it claims that its H100-based machines are faster than Instinct MI300X-powered servers.
Nvidia claims that AMD did not use optimized software for the DGX H100 machine, used to compare the performance to its Instinct MI300X-based server. Nvidia notes that high AI performance hinges on a robust parallel computing framework (which implies CUDA), a versatile suite of tools (which, again, implies CUDA), highly refined algorithms (which implies optimizations), and great hardware. Without any of the aforementioned ingredients, performance will be subpar, the company says.
According to Nvidia, its TensorRT-LLM features advanced kernel optimizations tailored for the Hopper architecture, a crucial performance enabler for its H100 and similar GPUs. This fine-tuning allows for models such as Llama 2 70B to run accelerated FP8 operations on H100 GPUs without compromising precision of the inferences.
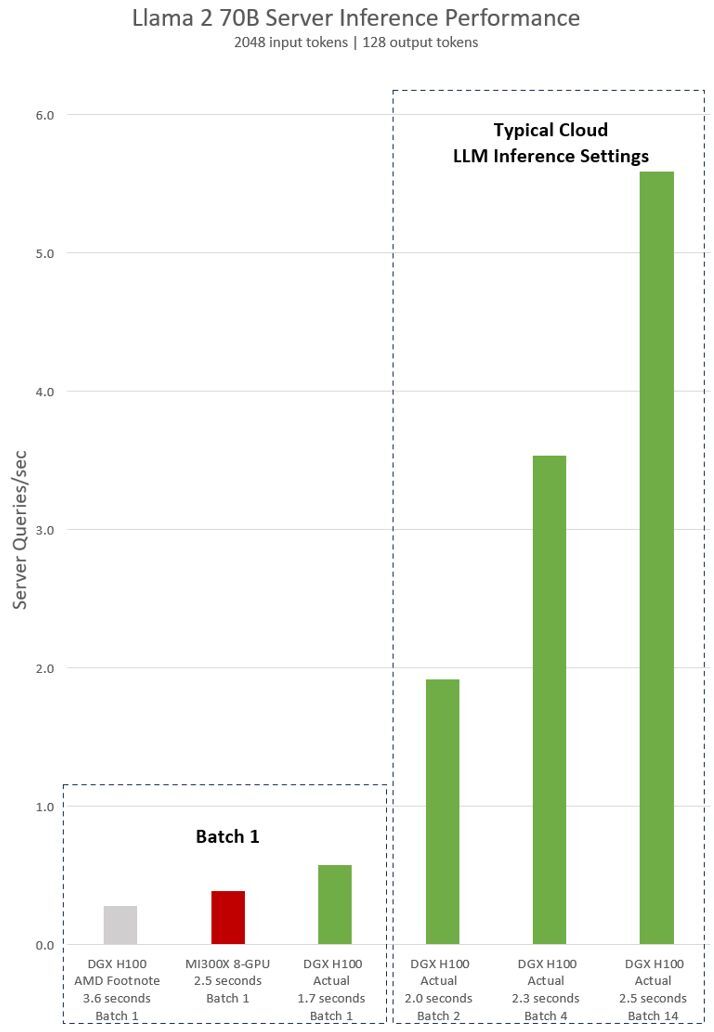
To prove its point, Nvidia presented performance metrics for a single DGX H100 server equipped with eight H100 GPUs running the Llama 2 70B model. A DGX H100 machine is capable of completing a single inference task in just 1.7 seconds when set to a batch size of one, meaning it handles one request at a time, which is lower compared to AMD's eight-way MI300X machine's 2.5 seconds (based on the numbers published by AMD). This configuration provides the quickest response for model processing.
However, to balance response time and overall efficiency, cloud services often employ a standard response time for certain tasks (2.0 seconds, 2.3 seconds, 2.5 seconds on the graph). This approach allows them to handle several inference requests together in larger batches, thereby enhancing the server's total inferences per second. This method of performance measurement, which includes a set response time, is also a common standard in industry benchmarks like MLPerf.
Even minor compromises in response time can significantly boost the number of inferences a server can manage simultaneously. For instance, with a predetermined response time of 2.5 seconds, an eight-way DGX H100 server can perform over five Llama 2 70B inferences every second. This is a substantial increase compared to processing less than one inference per second under a batch-one setting. Meanwhile, Nvidia naturally did not have any numbers for AMD's Instinct MI300X when measuring performance in this setup.