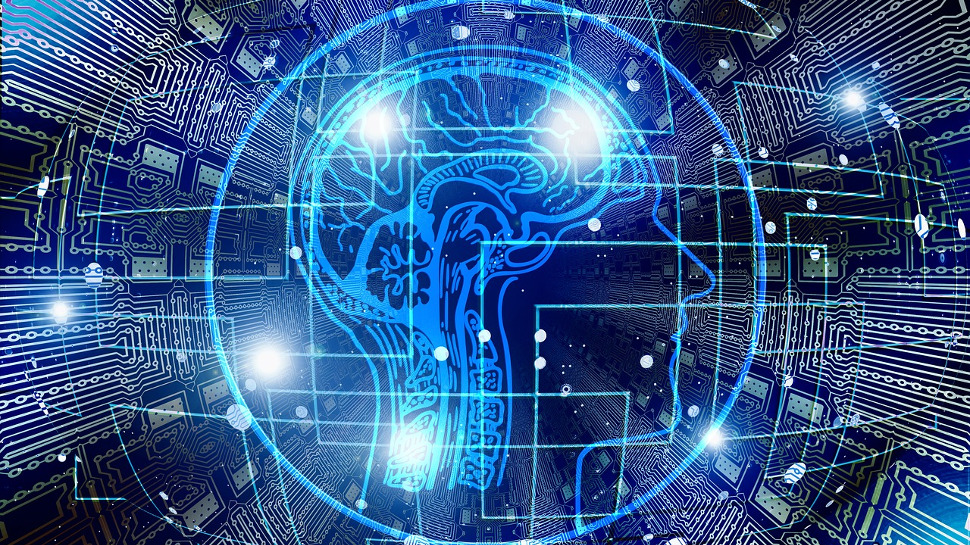
The momentum of AI-driven applications is accelerating around the world and shows little sign of slowing. According to data from IBM, 42% of companies with more than 1000 employees are actively using AI in their business, and a further 40% are trialing and experimenting with it.
As AI adoption gains pace, with platforms such as OpenAI’s GPT-4o and Google’s Gemini setting new benchmarks of performance, organizations are discovering new applications for these technologies that can deliver better outcomes. Faced with new challenges of deploying the technology at scale. More and more enterprise workflows are embedding calls to these AI Models are dramatically increasing usage. Do the use cases justify the escalating spending on the latest models?
Embracing AI also means embracing the usage of AI models and paying for AI inference costs, at a time when many organisations are in cost-cutting mode. With continued economic uncertainty, rising operational costs, and increasing pressure from stakeholders to deliver ROI, businesses are looking for ways to optimize their budgets and reduce unnecessary expenditures. The escalating costs of AI infrastructure can be a cause of tension, as organizations want to remain competitive and leverage the power of AI, while also finding the balance between these investments and financial prudence.
To further complicate things, AI agents which, according to McKinsey are the next frontier of GenAI and are largely expected to form the next wave of applications, will dramatically increase usage of AI models as they rely on them for ongoing reflection and planning steps. Instead of singular API calls to underlying models like those from OpenAI, agentic architectures can make scores of calls, thus racking up those costs. How can businesses navigate the rising costs of data while powering the AI applications they need?
Understanding the cost of AI at scale
The rapid deployment of AI is leading to increased costs on multiple fronts. First and foremost, organizations are spending on AI inference which is the process of using a trained model to make predictions or decisions based on provided inputs. Often, they would rely on APIs from the leading providers such as OpenAI, Anthropic or cloud service providers like AWS or Google and would pay based on usage. Alternatively, some organizations run their own inference and buy or rent GPUs on which they deploy open source models such as Llama from Meta.
Secondly, in many cases organizations want to customize their AI models by ‘fine-tuning’ them. This can at times be an expensive process involving data preparation by creation of training datasets and require compute resources for training.
Finally, building AI applications requires additional components, such as vector databases, which help augment inference by helping retrieve relevant content from designated knowledge bases and thus improve accuracy and relevance of responses from AI models.
By examining the root causes and the drivers of their AI costs such as AI inference, training or fine-tuning and additional components such as databases, businesses can minimize storage costs and enhance their AI application performance.
Optimizing efficiency through semantic caching
Semantic caching is a highly effective technique that organizations are deploying to manage the cost of AI inference and to increase speed and responsiveness of their applications. It refers to storing and reusing the results of previous computations based on their semantic meaning.
In other words, instead of relying on new AI computations for new queries, a semantic cache can check a database for queries with similar meanings that have been asked before, thus saving costs. This approach helps reduce redundant computations and improves efficiency in applications like inference or search.
In one particular study, researchers showed that up to 31% of queries to AI applications can be repetitive. Every unnecessary AI inference call adds avoidable costs, but by implementing a semantic cache, organizations can significantly reduce these calls, cutting them by 30-80%. This method is crucial for building scalable and responsive generative AI applications or chatbots. This approach not only optimizes cost but also accelerates response times, helping businesses achieve more with less investment.
Balancing performance and cost
Organisations need to optimise their technology stack and operational strategies to be able to deploy cutting-edge AI applications without incurring unsustainable infrastructure costs. This can help them strike that crucial balance between performance and cost. Techniques such as semantic caching can play a vital role in this.
For companies that are struggling with scaling AI applications in an efficient and cost-effective way, learning how to effectively manage this would become a key market differentiator. The key to businesses navigating the spiraling cost of generative AI applications and maximizing their value could lie in the AI Inference strategy. As generative AI systems get more and more complex, every LLM call needs to be as efficient as possible. By doing so, customers can get to the information they need faster and businesses can minimize their cost footprint.
We've featured the best AI website builder.
This article was produced as part of TechRadarPro's Expert Insights channel where we feature the best and brightest minds in the technology industry today. The views expressed here are those of the author and are not necessarily those of TechRadarPro or Future plc. If you are interested in contributing find out more here: https://www.techradar.com/news/submit-your-story-to-techradar-pro