The 2024 Nobel prize in chemistry has been awarded to three scientists for their work on describing and predicting proteins with the help of computers. One half of the prize goes to David Baker from the University of Washington in the US “for computational protein design”, with the other half jointly awarded to Demis Hassabis and John M. Jumper, both from Google Deepmind, UK, “for protein structure prediction”.
Using computers to carry out protein design and for predicting protein structures are two sides of the same coin. They are separately very powerful – and combined, even more so.
Proteins are the building blocks of life, building and powering our muscles and organs. Proteins are molecular machines: they read and copy our DNA to make new cells, and pump ions (electrically charged atoms or groups of atoms) into and out of our cells, so these always have what they need to work properly. Proteins act as sensors, detecting what’s in their environment. They also activate our immune systems.
The molecular building blocks of proteins are amino acids. These connect, one end to another, like letters joining to form a word. Exactly like a word, scientists give a letter to each amino acid, and these can spell out any given protein.
Just having that protein sequence – the “word” – isn’t enough, though. It’s the three-dimensional shape of the protein that determines how it works. So, if we want to make a protein for some purpose, we need a way to determine what its three-dimensional shape will be from the amino acid sequence alone. This is protein structure prediction.
Some proteins can be prepared in such a way that their structure can be determined by X-ray, but most cannot. This is why computational structure prediction is vitally important.
It is still an extraordinarily difficult problem. Even a small protein, of around 100 “letters” or amino acids, has an impossibly high number of possible ways it can be arranged in three dimensions. To visualise this, imagine arranging strands of cooked spaghetti in a bowl.
Read more: Nobel Prize in physics spotlights key breakthroughs in AI revolution − making machines that learn
For this reason, until the last decade, computational structure prediction had very low accuracy – less than 50%, in fact. Then, in 2020, Hassabis and Jumper developed an AI tool called AlphaFold2. This can predict the three-dimensional structure of a protein, using only the sequence of letters, with over 90% accuracy.
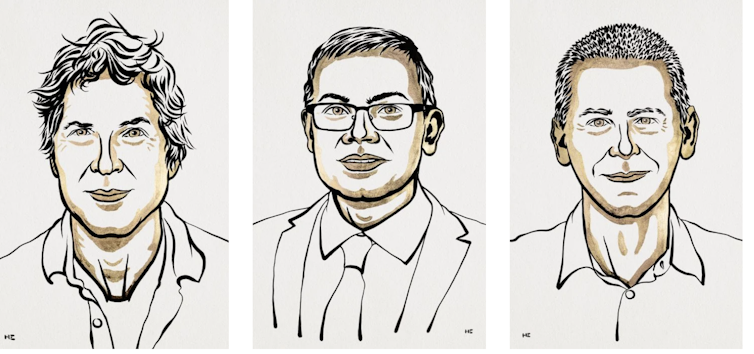
To make such a leap in accuracy, AlphaFold2 uses deep learning and neural networks. Deep learning is a computer-based approach that simulates the way the human brain makes decisions. Neural networks mimic the human brain’s structure and function to process data.
AlphaFold2 also makes use of massive databases of known protein structures and sequences. The neural network correlates the known three-dimensional shapes with the amino acid sequence. It can then derive rules for what shape a given sequence – the “letters” – will adopt.
The opposite problem, computational protein design, can be summed up by the following question: “I want a protein with this three-dimensional shape; what is the sequence that gives me that shape?”
This challenge was actually solved first. In 2003, Baker wrote a computer program called Rosetta that begins with the desired three-dimensional structure, and produces the amino acid sequence that will give that structure. It uses the idea that the three-dimensional structure of the entire protein can be built from the structures of small fragments.
Applying the science
Computational protein design has many applications. Proteins have been designed to bind and inactivate viruses, to detect drugs like fentanyl, and even to degrade plastic in the environment.
So, why has this prize been awarded for these advances now? Protein design and prediction are both inherently complex problems. There is no way to shortcut the large number of possible structures. But the rapid rise in the capabilities and use of artificial intelligence methods has given us a way to address this complexity. AI can efficiently derive correlations from millions of protein structures.
The pace of development in AI approaches is highlighted by this year’s Nobel prize in physics, which was awarded for the development of neural networks.
The twin methods of computational protein design and computational protein structure prediction are now real tools, used by millions of scientists worldwide. Proteins to counter pandemic viruses can now be designed in a matter of weeks.
It therefore wouldn’t be surprising if we see many other Nobels in future being awarded for breakthroughs that use the power of artificial intelligence.

Matthew Addicoat receives funding from EPSRC and the Royal Society.
This article was originally published on The Conversation. Read the original article.