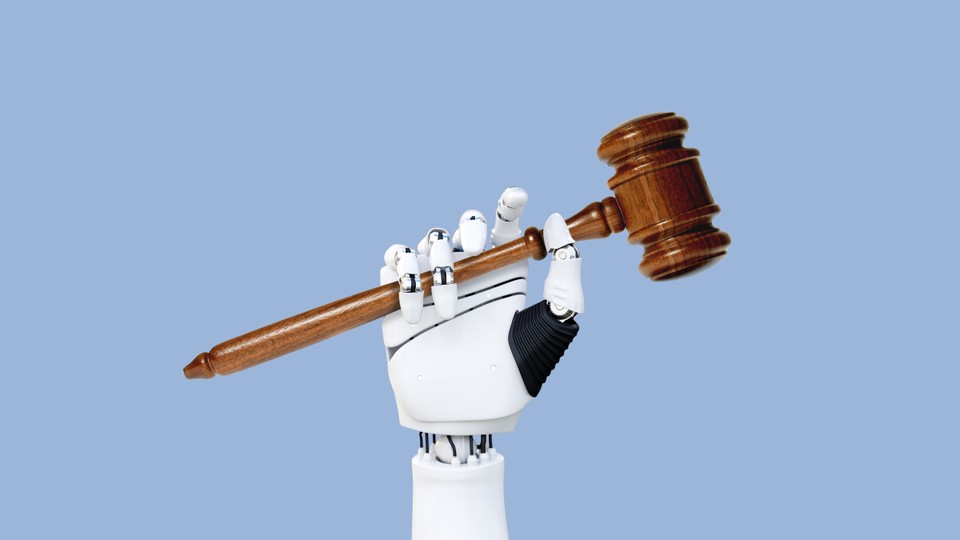
The system for granting asylum in the U.S. has long been a political point of contention. Democrats and Republicans debate how liberal or restrictive its rules should be, but evidence suggests that the fate of some asylum seekers may be less influenced by the rules than by something far more arbitrary: the judge they’re assigned.
A 2007 study titled “Refugee Roulette” found that one judge granted asylum to only 5 percent of Colombian applicants, whereas another—working in the same building and applying the same rules—granted it to 88 percent.
Asylum is by no means the only part of our legal system where such discrepancies arise. In a landmark 1974 study, 50 judges were given an identical set of facts about a hypothetical heroin dealer. Their proposed sentences ranged anywhere from one to 10 years. The problem persists today. A series of more recent studies suggests that some judges show greater leniency toward defendants of their own race, whereas others are systematically harsher on such defendants.
This kind of variability—the kind that comes from any exercise of human judgment—creates massive unpredictability in the legal system. Because humans are inconsistent and subjective, we’ve come to see this as a fixed feature of our justice system. But it doesn’t have to be. Artificial intelligence, used in concert with human decision making, offers us a way to rein in the inconsistency that distorts the application of our laws and subjects so many people to unpredictable judgments.
Some variability is to be expected, even desired: Our legal ideals— fairness, justice, proportionality—lend themselves to a range of reasonable interpretations. And judicial discretion is crucial for preserving the rule of law; without it, those with political power could bend judicial outcomes to their will. This is why courts controlled by nondemocratic leaders are likened to kangaroos: They “jump” over evidence, reason, and rules to get to a preordained result.
But just how wide a range of judicial outcomes is acceptable? When a law’s impact is primarily determined not by the facts of a case or even by the law itself, but by the person applying it, our entire system of democratic lawmaking suffers.
Judges who are free from external meddling are nevertheless subject to a series of internal threats in the form of political prejudice, inaccurate prediction, and cognitive error. In their book Noise, Daniel Kahneman, Cass Sunstein, and Olivier Sibony show that these threats come in two flavors: bias and noise. Bias is a systematic leaning in a particular direction—when the readings of a scale are always too low or too high. Noise is unpredictability in all directions—when the results are always different.
Each time we statistically analyze legal decisions, we seem to find more evidence of bias and noise. Defendants are shown more leniency on their birthday, for example; judges are more likely to deny parole the longer they’ve gone without a food break, and they hand out harsher decisions after their local sports team loses.
Of course, judges are skilled at rationalizing their conclusions. But just because you’d never find these influences mentioned in their judgments does not make them any less real.
For our justice system to remain responsive to democratic lawmaking and live up to our legal ideals, the judgments it produces must fall within a reasonably limited range. In other words, to ensure the integrity of the law, we need not only judicial autonomy but also reasonable predictability. That’s where artificial intelligence can help.
AI has many potential applications in the justice system. Imagine, for instance, a judge tasked with determining whether a defendant represents a flight risk, or whether the use of a particular brand or logo will “likely cause confusion” with a registered trademark. Statistics could quantify these determinations with much larger data sets than those that judges have access to. AI could also help stamp out prejudice by showing us when and how systemic biases give undue influence to criteria such as race, gender, and ethnic origin.
In such cases—and many more—less humanity could lead to more fairness.
Entrusting justice to technology will understandably cause some alarm. For one thing, many data sets are already contaminated by historical prejudice. The discriminatory decisions of the past may cause certain data to suggest that being a member of a disadvantaged group constitutes a risk factor in and of itself (for example, in decisions that evaluate the risk of recidivism in bail hearings). If we aren’t careful, AI could end up entrenching discrimination instead of reducing it.
To mitigate this risk, AI and human judgment must work together, each alleviating the potential biases of the other. Any decision AI makes, no matter how minor, should be subject to human review.
We will never be able to achieve a perfect ideal of fairness, but AI can help us achieve something measurably fairer than our current system. To take self-driving cars as an analogy: The correct safety benchmark isn’t whether they’ll lead to no accidents but whether they’ll lead to significantly fewer accidents than human drivers.
Integrating AI into the justice system should be done gradually. One relatively modest application would be for AI to notify judges when their determinations fall outside a standard range of outcomes in comparable cases. This would offer judges an opportunity to self-correct without imposing any direct constraint on their decision making.
Furthermore, AI-driven analysis could open new avenues of appeal to a defendant when his or her punishment is determined to be abnormally stringent. A somewhat more assertive application could be using AI to refine mandatory minimums and statutory maximums by reviewing past judgments. Fine-tuning these applications will be the difference between merely reinforcing the decision-making tendencies of past deciders and actually reducing bias and noise.
Justice may be blind, but human beings are fallible. Our thinking is clouded by more prejudices than we can count, not to mention an excessive confidence in our own judgment. A fairer legal system may need to be a little less human.