OpenAI, developer of ChatGPT and a leading innovator in the field of artificial intelligence (AI), was recently thrown into turmoil when its chief-executive and figurehead, Sam Altman, was fired. As it was revealed that he would be joining Microsoft’s advanced AI research team, more than 730 OpenAI employees threatened to quit. Finally, it was announced that most of the board who had terminated Altman’s employment were being replaced, and that he would be returning to the company.
In the background, there have been reports of vigorous debates within OpenAI regarding AI safety. This not only highlights the complexities of managing a cutting-edge tech company, but also serves as a microcosm for broader debates surrounding the regulation and safe development of AI technologies.
Large language models (LLMs) are at the heart of these discussions. LLMs, the technology behind AI chatbots such as ChatGPT, are exposed to vast sets of data that help them improve what they do – a process called training. However, the double-edged nature of this training process raises critical questions about fairness, privacy, and the potential misuse of AI.
Training data reflects both the richness and biases of the information available. The biases may reflect unjust social concepts and lead to serious discrimination, the marginalising of vulnerable groups, or the incitement of hatred or violence.
Training datasets can be influenced by historical biases. For example, in 2018 Amazon was reported to have scrapped a hiring algorithm that penalised women – seemingly because its training data was composed largely of male candidates.
LLMs also tend to exhibit different performance for different social groups and different languages. There is more training data available in English than in other languages, so LLMs are more fluent in English.
Can companies be trusted?
LLMs also pose a risk of privacy breaches since they are absorbing huge amounts of information and then reconstituting it. For example, if there is private data or sensitive information in the training data of LLMs, they may “remember” this data or make further inferences based on it, possibly leading to the leakage of trade secrets, the disclosure of health diagnoses, or the leakage of other types of private information.
LLMs might even enable attack by hackers or harmful software. Prompt injection attacks use carefully crafted instructions to make the AI system do something it wasn’t supposed to, potentially leading to unauthorised access to a machine, or to the leaking of private data. Understanding these risks necessitates a deeper look into how these models are trained, the inherent biases in their training data, and the societal factors that shape this data.
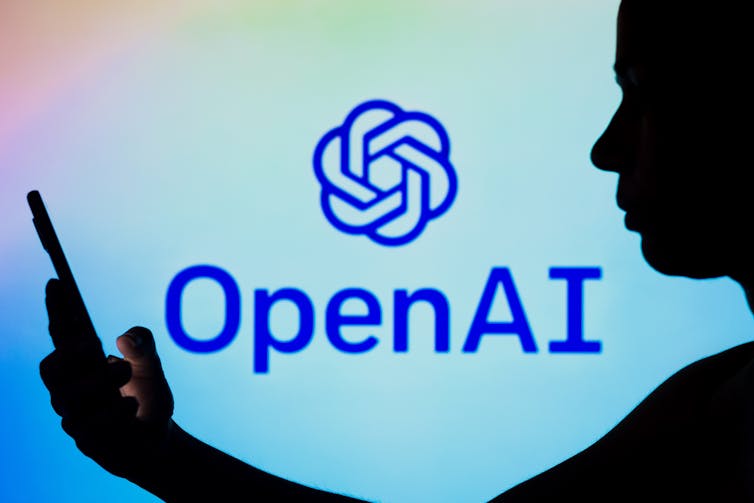
The drama at OpenAI has raised concerns about the company’s future and sparked discussions about the regulation of AI. For example, can companies where senior staff hold very different approaches to AI development be trusted to regulate themselves?
The rapid pace at which AI research makes it into real-world applications highlights the need for more robust and wide-ranging frameworks for governing AI development, and ensuring the systems comply with ethical standards.
When is an AI system ‘safe enough’?
But there are challenges whatever approach is taken to regulation. For LLM research, the transition time from research and development to the deployment of an application may be short. This makes it more difficult for third-party regulators to effectively predict and mitigate the risks. Additionally, the high technical skill threshold and computational costs required to train models or adapt them to specific tasks further complicates oversight.
Targeting early LLM research and training may be more effective in addressing some risks. It would help address some of the harms that originate in training data. But it’s important also to establish benchmarks: for instance, when is an AI system considered “safe enough”?
The “safe enough” performance standard may depend on which area it’s being used in, with stricter requirements in high-risk areas such as algorithms for the criminal justice system or hiring.
Read more: AI will soon become impossible for humans to comprehend – the story of neural networks tells us why
As AI technologies, particularly LLMs, become increasingly integrated into different aspects of society, the imperative to address their potential risks and biases grows. This involves a multifaceted strategy that includes enhancing the diversity and fairness of training data, implementing effective protections for privacy, and ensuring the responsible and ethical use of the technology across different sectors of society.
The next steps in this journey will likely involve collaboration between AI developers, regulatory bodies, and a diverse sample of the general public to establish standards and frameworks.
The situation at OpenAI, while challenging and not entirely edifying for the industry as a whole, also presents an opportunity for the AI research industry to take a long, hard look at itself, and innovate in ways that prioritise human values and societal wellbeing.

Yali Du does not work for, consult, own shares in or receive funding from any company or organisation that would benefit from this article, and has disclosed no relevant affiliations beyond their academic appointment.
This article was originally published on The Conversation. Read the original article.