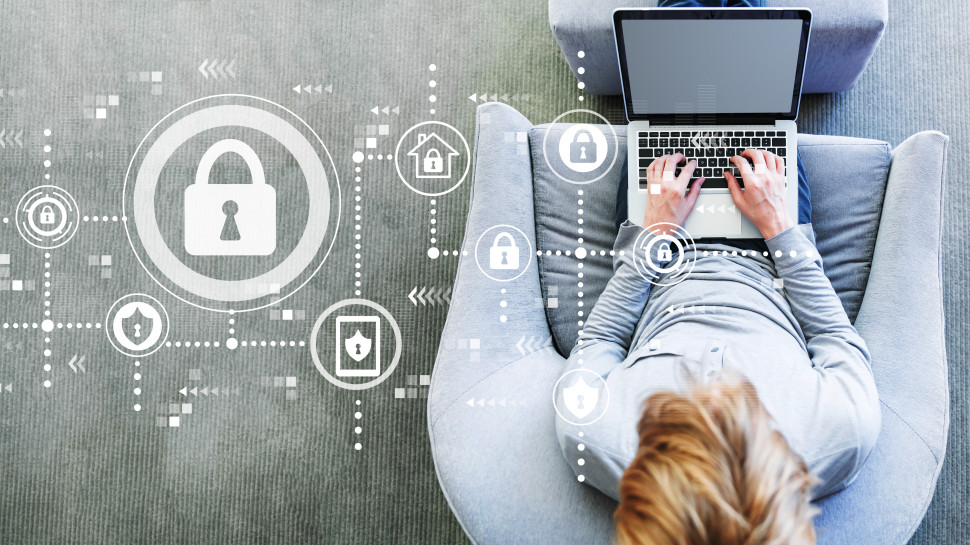
One of the four key themes at the World Economic Forum this year in Davos was “Artificial Intelligence (AI) as a Driving Force for the Economy and Society.” There were between 10-15 sessions that at least touched on AI, if not focused exclusively on this highly influential technology. While many of these panels stressed the potential benefits of generative AI and large language models (LLM) to industries such as fintech, health research, and climate science, an even greater emphasis was placed on concern over AI’s pervasive reach into our personal lives and the potential ramifications.
For AI to be effective, it requires massive amounts of data from which it trains itself. The positive outputs of this include deep learning models that can recognize complex patterns to produce accurate predictions, for example, enabling biometrics for homeland security and financial fraud protection. The most prevalent use today of emerging AI is in big data algorithms for targeted ads and real-time translation applications, which continuously improve via further enlarged pools of data.
However, a line must be drawn between publicly available information to train AI systems and personal and proprietary data that is increasingly being exploited and analyzed without user consent and despite being of sensitive nature. One example for this is biometric security, which, while great for securing our borders, is also highly personal and can easily be used nefariously if it falls into the wrong hands.
This raises another topic of concern with AI: the potential for leaks and breaches. Unfortunately, most existing AI and LLM platforms and apps (such as ChatGPT) are riddled with vulnerabilities, so much so that many large enterprises have banned their use to protect their proprietary secrets, a trend we see gaining scale and scope.
Therefore, among the prevalent topics at Davos was also the dire need for regulation and limiting AI’s reach, present and future, especially as it pertains to privacy. There is already much data-related regulation in place, such as HIPAA, GDPR, and CCPA/CPRA, but such legislation requires companies to be transparent about their usage of private information, or it enables consumers to opt-out of programs that would otherwise use their personal data. That is effective in terms of encouraging accountability, but regulation and policy cannot actually protect data from leaks or vectored attacks.
Challenges in secure data processing
The only means of truly securing our privacy is through proactive enforcement of the utmost secure and novel technological measures at our disposal, those that ensure a strong emphasis on privacy and data encryption, while still enabling breakthrough technologies such as generative AI models and cloud computing tools full access to large pools of data in order to meet their full potential.
Protecting data when it is at rest (i.e., in storage) or in transit (i.e., moving through or across networks) is ubiquitous. The data is encrypted, which is generally enough to ensure that it remains safe from unwanted access. The overwhelming challenge is how to also secure data while it is in use (i.e., being processed and analyzed).
The leading privacy-enhancing technology in use today at scale is Confidential Computing, which attempts to safeguard a company’s IP and sensitive data by creating a dedicated enclave, called a Trusted Execution Environment (TEE), in the server CPU wherein sensitive data is processed. Access to the TEE is restricted to ensure that when the data therein is decrypted for processing, it is inaccessible by any of the compute resources beyond the ones being used within the TEE.
One major issue with Confidential Computing is that it cannot scale sufficiently to cover the magnitude of use cases necessary to handle every possible AI model and cloud instance. Because a TEE must be created and defined for each specific use case, the time, effort, and cost involved in protecting data is restrictive.
The bigger issue with Confidential Computing, though, is that it is not foolproof. The data in the TEE must still be unencrypted for it to be processed, opening the potential for quantum attack vectors to exploit vulnerabilities in the environment. If the data is decrypted at any point in its lifecycle, it is potentially exposed. Moreover, when the AI or computing tools are accessing personal data, even in the TEE, all anonymity has been lost once it is decrypted.
Revolutionizing data privacy
The only post-quantum technology for privacy is lattice-based Fully Homomorphic Encryption (FHE), which enables processing of data while it remains encrypted throughout its lifecycle, including while it is being processed. This ensures that there can be no leakage and no data breaches, and it ensures anonymity of the data that is in use.
The benefits of FHE are felt both in the efficacy of the AI and cloud computing tools and in the assurance of security for individuals and the companies tasked with protecting their data. For example, imagine how much more effective an AI model for early detection of cancer can be when it has access to millions of patient records instead of thousands. And yet, every one of those records remains securely encrypted, ensuring that they cannot be breached or leaked, and not a single patient is known to the model. Confidentiality is, therefore, maintained at every point in time.
The one hurdle that has thus far restricted FHE from being adopted and used at scale, is the massive processing burden it entails to overcome profound bottlenecks in memory, compute, and bandwidth. It is estimated that implementing FHE across a hyperscale cloud data center would necessitate one million times acceleration over today’s latest generation CPUs and GPUs. A growing number of software-based solutions have surfaced in recent years, though they struggle to scale enough to meet the demands of machine learning, deep learning, neural networks, and compute heavy algorithm operations on the cloud.
Only a dedicated architecture can address these specific bottlenecks and make real-time FHE possible at a TCO that is on par with that for processing unencrypted data, such that the end user cannot tell the difference between processing on a CPU or any other type of processor. As such, it grows increasingly obvious why Sam Altman, CEO of OpenAI, is investing $1 billion into developing a dedicated hardware processor for private LLMs, and the hyperscale cloud service providers are following suit.
Privacy: the next frontier
Now that generative AI has emerged as a centerpiece of Davos and other global forums, it is rightfully garnering the attention it deserves, both for its potential benefit to society and its shortcomings. Any analysis of the challenges AI poses will inevitably land on privacy as the poster child.
Thus, privacy is fast becoming the next massive technology industry. As ever more technological breakthroughs surface that capitalize on our personal data, and as data is created and processed at an exponential rate, the greater the demand for security measures to ensure our privacy.
Regulation cannot protect us. Only a technological solution can address a technological problem. And for privacy, only a dedicated post-quantum solution will prevail.
We've featured the best business VPN.
This article was produced as part of TechRadarPro's Expert Insights channel where we feature the best and brightest minds in the technology industry today. The views expressed here are those of the author and are not necessarily those of TechRadarPro or Future plc. If you are interested in contributing find out more here: https://www.techradar.com/news/submit-your-story-to-techradar-pro