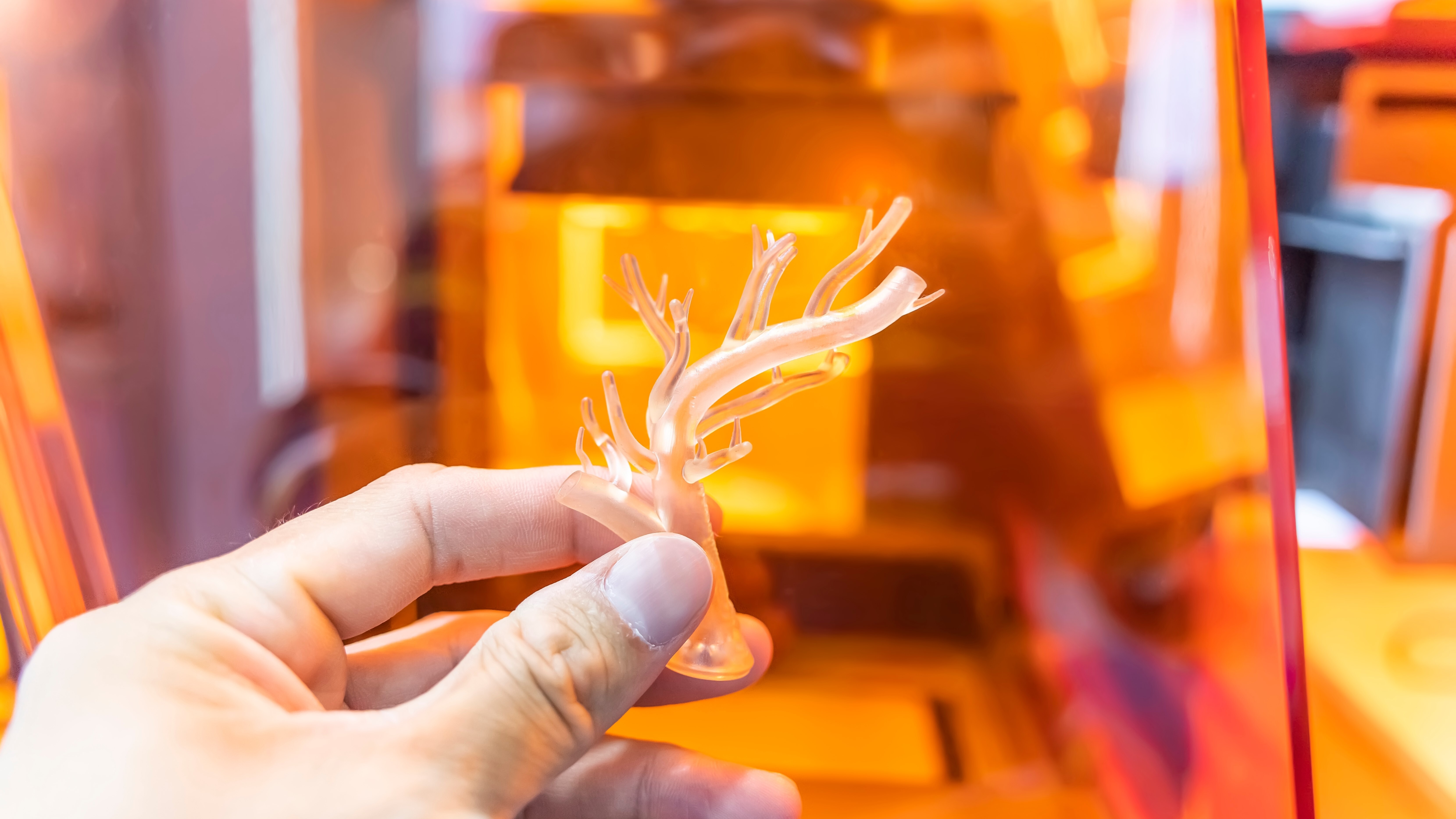
Researchers from the Washington State University (WSU) School of Mechanical and Materials Engineering developed an AI technique called Bayesian Optimization to find the optimum 3D printing settings the team needed to recreate lifelike 3D-printed models of human organs. While this may sound simple, the group needed to determine a multitude of variables to find the best solution, and finding the best setup could take a lot of time.
These include looking for the most suitable materials, 3D printing configurations, nozzle pressure, and more. “The sheer number of potential combinations is overwhelming, and each trial costs time and money,” Associate Professor Jana Doppa of Computer Science at WSU told Tech Xplore.
So, to save time, effort, and materials, the WSU research team, led by Kaiyan Qui, Assistant Profession in the WSU School of Mechanical and Materials Engineering, and Prof. Doppa, trained an AI model to optimize the 3D printing process of organ models used in surgeon training and implant evaluation. The AI looked at three crucial characteristics: the model’s geometric precision, its density, and printing time.
By balancing all three, the team could 3D print organs that closely resemble the look and feel of an actual human organ without taking too long to produce. Aside from ensuring that the parts of the organ, like veins and arteries, are accurately represented, the correct density also ensures that the 3D-printed organ feels as close as possible to the actual tissue.
“It’s hard to balance all the objectives, but we were able to strike a favorable balance and achieve the best possible printing of a quality object, regardless of the printing type or material shape,” Eric Chen, a WSU visiting student who worked with Qui, said to Tech Xplore. This allowed the team to 3D print a model of a prostate for use in surgical rehearsal. The group also used the same AI to 3D print a realistic model of a kidney after a few minor changes in the code.
Beyond that, the AI model can also be used in other fields like automotive engineering and aviation with just the proper tweaks. This could potentially turbocharge manufacturing and design, allowing factories to optimize their designs with less effort. This, in turn, could lead to cheaper products that use materials much more efficiently.