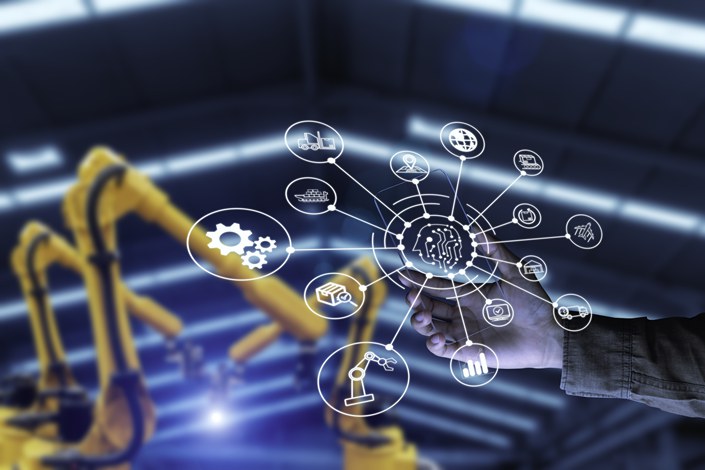
Human activities are changing Earth’s climate. By making more accurate predictions and using resources more efficiently, artificial intelligence (AI) has the potential to help reduce climate change. By contrast, the training and use of large deep learning (DL) models is not energy-efficient, and sometimes even contributes adversely to climate change.
Climate change is one of the greatest challenges facing humanity in the 21st century. All nations must make extreme efforts to reduce emissions to achieve the two-degree target for global temperature rises set in Dec. 2015 as part of the Paris Agreement. In addition to converting energy supplies to renewable sources of energy, technical innovations play a major role in reducing and avoiding emissions.
To achieve these goals, great hopes are being placed in AI, as it has great potential to make all kinds of processes both more efficient and energy-saving. AI looks promising in its ability to make more accurate predictions and to accelerate the advancement and widespread adoption of new technologies like nuclear fusion. Some 80 percent of all world-wide emissions are energy-related, and thus there is enormous unexploited potential, especially in the energy industry, for saving energy with the help of AI.
AI Apps’ Efficient Resource Use
For example, AI can help find optimal locations for renewable energies, improve wind power plants’ use of accurate weather forecasts, and detect potential problems in smart grids before they occur. With future-oriented topics such as autonomous driving, AI is already present today, especially in the mobility sector. In addition, there are several other cases in which AI is already being successfully deployed, such as the coordination of groupage freight, dynamic pricing, intelligent route finding, and automated traffic control.
AI is likewise already being used in many areas of agriculture. Livestock farming and fertilizer use are the two main factors driving carbon dioxide (CO2) emissions in this industry. To help reduce these emissions, precision farming technologies can be used to calculate the ideal nutrient and fertilizer use for individual plants, thus increasing yields on the one hand, while also better protecting the environment on the other.
AI is also used in agricultural robotics, weather forecasts and early warning systems for plant infections. Due to increasing urbanization and higher concentrations of people, businesses, and traffic, cities have become the biggest CO2 emitters, but they also offer enormous opportunities for savings. This means that there is potential for emissions to be quickly reduced, especially through intelligent control of buildings and automated traffic management. In addition, data analyses can help us make decisions on sustainable infrastructure measures and related investments.
Severer Legal Challenges
In the areas mentioned above, there are often major technical and legal hurdles in the collection and exchange of data. Furthermore, the AI Act proposed by the European Commission would unnecessarily classify most AI applications as high-risk. Consequently, most of them would then further face unfulfillable legal requirements, demand complex governance, and make data collection and sharing even more difficult.
Large DL Models Are Unsustainable
AI as an interdisciplinary technology promises to enable unexploited savings potential for the reduction of emissions in many areas. It is important to note, however, that rebound effects could actually have the opposite effect. This means that the long-term sustainable application of the technology is crucial for AI to be able to make its desired contribution to the fight against climate change. In recent years, many achievements in AI, such as Generative Pre-Trained Transformer 3 in natural language processing, or You Only Look Once in computer vision, are largely based on DL.
Given the large and increasing number of AI experts, research labs and corporations working on AI, every few months there are substantially larger models for those tasks that keep being published and released. However, training and using them comes with an even larger ecological footprint. For example, it was shown just a few years ago that training a single DL model can generate up to 626,155 pounds of CO2 emissions, which is roughly equivalent to the total lifetime carbon footprint of five cars. One should also bear in mind that the research and development of such models may take many iterations, and the training of many versions of a model can result in skyrocketing costs and emissions. Given the large amount of electricity needed, the training of those DL models may end up costing millions of US dollars. In contrast, the human brain can learn extremely well from just a few instances, and requires very little energy.
DL is No Silver Bullet
It is also important to highlight the fact that deep neural networks are just one of many modern machine learning (ML) techniques. It is also becoming increasingly apparent that there is increasing hype surrounding deep learning, with more and more unrealistic promises being made about it. It is therefore essential to relate the successes of deep learning to its fundamental limitations. The no free lunch theorem, which is largely unknown in both industry and academia, states that all possible ML models averaged over all possible problems are equally accurate. Of course, some models are better suited to tackling some problems than others, but the trade-off is that they often perform worse on different problems.
DL is especially useful for many image, audio, or video processing-related problems, as well as when having much training data. In contrast, DL is often poorly suited to problems with only a small amount of training data. One should therefore not rely solely on DL models in AI projects, and instead start out with simpler models. In many projects, it is quite possible that these simpler models may turn out to not only solve a problem well, but also require far less energy and far fewer natural resources when trained and used. It is also crucial that one considers the impact and possible climate-related and social consequences of AI in a sustainable manner. AI developers should also be made aware of the emissions associated with their algorithms and realize that methods must be developed to reduce these.
Patrick Glauner is a professor of artificial intelligence at Deggendorf Institute of Technology in Germany.
The content herein is subject to copyright by The Yuan. All rights reserved. The content of the services is owned or licensed to Caixin Global. The copying or storing of any content for anything other than personal use is expressly prohibited without prior written permission from The Yuan, or the copyright holder identified in the copyright notice contained in the content.
The views and opinions expressed in this opinion section are those of the authors and do not necessarily reflect the editorial positions of Caixin Media.
If you would like to write an opinion for Caixin Global, please send your ideas or finished opinions to our email: opinionen@caixin.com
Get our weekly free Must-Read newsletter.