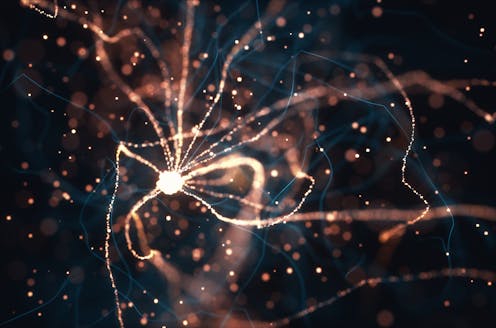
Understanding when and why a cell dies is fundamental to the study of human development, disease and aging. For neurodegenerative diseases such as Lou Gehrig’s disease, Alzheimer’s and Parkinson’s, identifying dead and dying neurons is critical to developing and testing new treatments. But identifying dead cells can be tricky and has been a constant problem throughout my career as a neuroscientist.
Until now, scientists have had to manually mark which cells look alive and which look dead under the microscope. Dead cells have a characteristic balled-up appearance that is relatively easy to recognize once you know what to look for. My research team and I have employed a veritable army of undergraduate interns paid by the hour to scan through thousands of images and keep a tally of when each neuron in a sample appears to have died. Unfortunately, doing this by hand is a slow, expensive and sometimes error-prone process.

Making matters even more difficult, scientists recently began using automated microscopes to continually capture images of cells as they change over time. While automated microscopes make it easier to take photos, they also create a massive amount of images to manually sort through. It became clear to us that manual curation was neither accurate nor efficient. Furthermore, most imaging techniques can detect only the late stages of cell death, sometimes days after a cell has already begun to decompose. This makes it difficult to distinguish between what actually contributed to the cell’s death from factors just involved in its decay.
My colleagues and I have been trying for some time to automate the curation process. Our initial attempts could not handle the wide range of cell and microscope types we use in our research, nor rival the accuracy of our interns. But a new artificial intelligence technology my research team developed can identify dead cells with both superhuman accuracy and speed. This advance could potentially turbocharge all kinds of biomedical research, especially on neurodegenerative disease.
AI to the rescue
Artifical intelligence has recently taken the field of microscopy by storm. A form of AI called convolutional neural networks, or CNNs, has especially been of interest because it can analyze images as accurately as humans can.
Convolutional neural networks can be trained to recognize and discover complex patterns in images. As with human vision, giving CNNs many example images and pointing out what features to pay attention to can teach the computer to recognize patterns of interest.
These patterns could include biological phenomena difficult to see by eye. For example, one research group was able to train CNNs to identify skin cancer more accurately than trained dermatologists. Even more recently, colleagues of mine were able to train CNNs to identify complex biological signatures such as cell type in microscopy images.
Building on this work, we developed a new technology called biomarker-optimized CNNs, or BO-CNNs, to identify cells that have died. First, we needed to teach the BO-CNN to distinguish between clearly dead and clearly alive cells. So we prepared a petri dish with mice neurons that were engineered to produce a nontoxic protein called a genetically encoded death indicator, or GEDI, that colored living cells green and dead cells yellow. The BO-CNN could easily learn that green meant “alive” and yellow meant “dead.” But it was also learning other features distinguishing living and dead cells that aren’t so obvious to the human eye.
After the BO-CNN learned how to identify the characteristics that distinguished the green cells from the yellow, we showed it neurons that weren’t distinguished by color. The BO-CNN was able to correctly label live and dead cells significantly faster and more accurately than people trained to do the same thing. The model could even look at images of cell types it had not seen before taken from different types of microscopes and still correctly identify dead cells.
One obvious question still remained, however – why was our model so effective at finding dead cells?
Researchers often treat the decisions CNNs make as black boxes, with the strategy the computer uses to solve a visual task considered less important than how well it performs. However, because there must be some patterns in the cell structure the model focuses on to make its decisions, identifying these patterns could help scientists better define what cell death looks like and understand why it occurs.
To figure out what these patterns were, we used additional computational tools to create visual representations of the BO-CNN’s decisions. We found that our BO-CNN model detects cell death in part by focusing on changing fluorescence patterns in the nucleus of the cell. This is a feature that human curators were previously unaware of, and it may be the reason designs for previous AI models were less accurate than the BO-CNN.
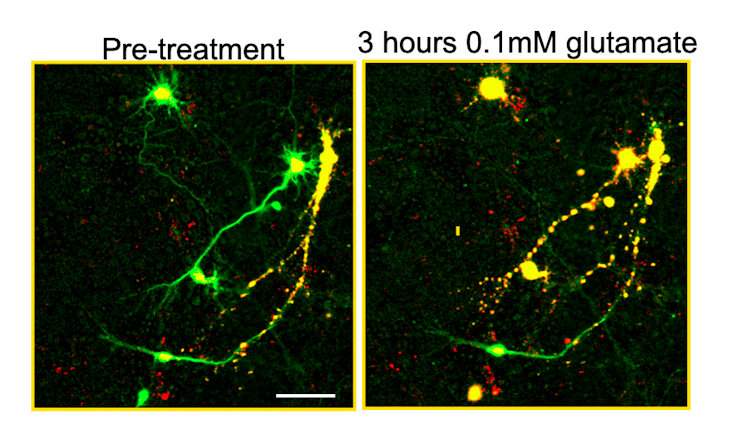
Harnessing the power of AI
I believe our approach represents a major advance in harnessing artificial intelligence to study complex biology, and this proof of concept could be broadly applied beyond detecting cell death in microscopic imaging. Our software is open source and available to the public.
Live-cell microscopy is extremely rich with information that researchers have difficulty interpreting. But with the use of technologies like BO-CNNs, researchers can now use signals from cells themselves to train AI to recognize and interpret signals in other cells. By taking out human guesswork, BO-CNNs increase the reproducibility and speed of research and can help researchers discover new phenomena in images that they would otherwise not have been able to easily recognize.
With the power of AI, my research team is currently working to extend our BO-CNN technology toward predicting the future – identifying damaged cells before they even start to die. We believe this could be a game-changer for neurodegenerative disease research, helping pinpoint new ways to prevent neuronal death and eventually lead to more effective treatments.
[Understand new developments in science, health and technology, each week. Subscribe to The Conversation’s science newsletter.]

Jeremy Linsley was supported by the National Institutes of Health (U54 NS191046, R37 NS101996, RF1 AG058476, RF1 AG056151, RF1 AG058447, P01 AG054407, U01 MH115747), the National Library of Medicine (R01 LM013617), the Koret Foundation, the Taube/Koret Center for Neurodegenerative Research, the National Center for Research Resources (RR18928), the Target ALS Foundation, the Amyotrophic Lateral Sclerosis Association Neuro Collaborative, Mike Frumkin, and the Department of Defense (W81XWH-13-ALSRP-TIA).
This article was originally published on The Conversation. Read the original article.