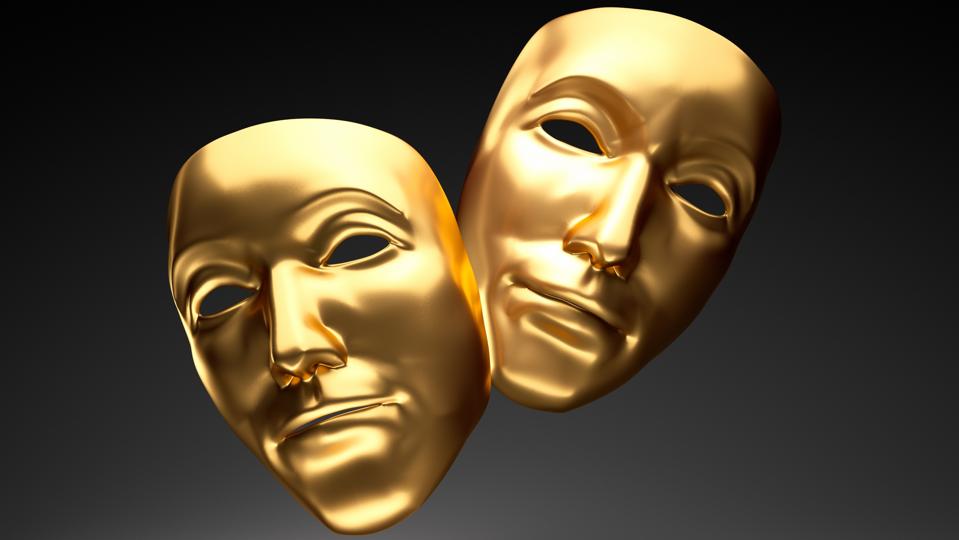
Fifty-one years ago, Apollo 13 was launched into space. Soon after, a tremendous explosion shook the spacecraft. With their precious oxygen supplies leaking into space, the three astronauts on board were in critical danger.
As the clock ticked, NASA’s Mission Control team in Houston worked non-stop. How could they diagnose and solve the challenges of a failing physical asset that was floating in space, 200,000 miles away?
Thankfully, NASA had 15 simulators that had trained the astronauts for the mission. These high-fidelity simulators with their computer systems were the predecessors of “digital twins.” They were used to replicate the damaged spacecraft and played a crucial role in the crew’s successful rescue.
Today, digital twins of human bodies are the latest trend in life sciences. Pharmaceutical companies are exploring this technology to transform drug development and patient care.
What are digital twins?
A digital twin is a virtual replica of a real-world person, physical entity, or process. For example, the digital twin of an aircraft engine is a precise virtual copy of the machinery, powered by artificial intelligence (AI). Streaming data collected from sensors onboard the engine is relayed to the digital twin in real-time. This enables aircraft engineers to monitor the engine’s performance and predict when it may fail.
Now, imagine building a digital twin of a real person to replicate how they would behave and respond in specific situations. You could track their health, diagnose diseases, and plan preventive treatments.
While a full-blown recreation of a human in the virtual world is still beyond possibility (thankfully!), even a partial replication offers significant benefits today.
Why are digital twins so important in healthcare? We could use them to test new drugs to ascertain drug safety and effectiveness. If we had access to such technology in 2020, COVID-19 vaccines could have arrived months earlier and saved millions of lives.
Why clinical trials need some disruption
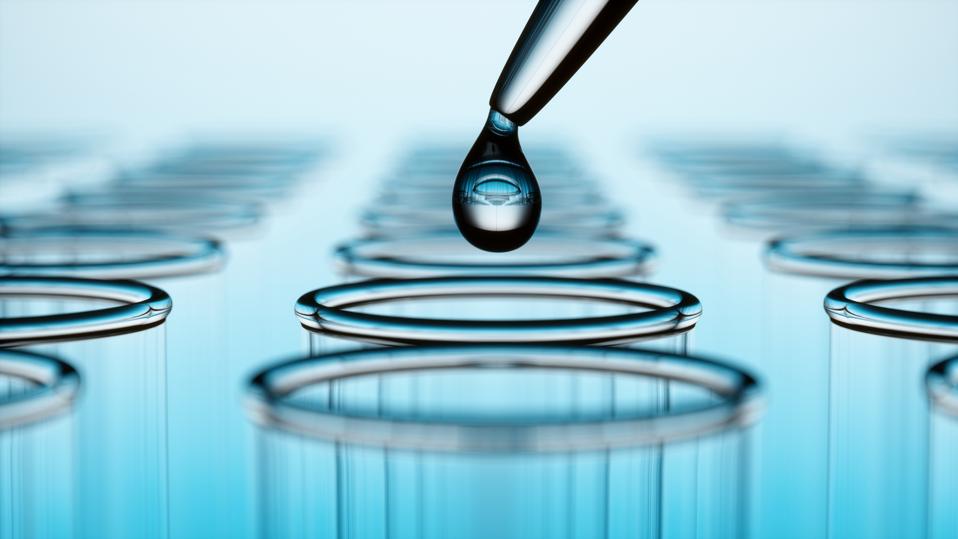
Today, four key challenges plague clinical trials and make drug development imperfect, slow, unpredictable, and even unsafe.
1. They’re not an accurate representation of the real world
By design, trials can factor only a tiny representation of the large and varied population in the real world. Of the billions of people on earth, how each one of them could react to a drug based on their unique physical and mental state can’t be recreated in clinical trials.
2. Few trials recruit the needed patients on time
Some clinical trials never produce results because the researchers aren’t able to recruit the right patients. Pharma companies struggle to onboard the specified number and type of patients to meet the stringent specifications of clinical trial designs. In fact, recruitment challenges delay almost 80% of all trials.
3. Not every patient is treated by a trial’s new drug
In most trials, the actual drug is swapped with a placebo or a dummy drug for up to half of the patients. This helps contrast how patients with the disease respond when the experimental drug is not given. This means that at least some patients who sign up for trials, hoping to receive a new treatment, do not get it.
4. Not all experimental drugs work as safely as intended
By design, clinical trials assess the safety and effectiveness of experimental drugs. Despite regulatory checks and controls to improve the safety of drug discovery, trials have severe adverse outcomes, including death. This dissuades many patients from signing up for clinical trials, and those who join do so amidst safety concerns.
Digital twins can address these challenges:
- Coverage: Digital twins can simulate a wide variety of patient characteristics, providing a representative view of a drug’s impact on a broader population.
- Speed: AI can simplify trial design by providing visibility into patient availability for a variety of inclusion and exclusion criteria.
- Predictability: With digital twins predicting patient response, there will be no need for placebos or dummy drugs. So, every patient in a trial can be assured of the new treatment.
- Safety: By reducing the number of patients who need real-world testing, digital twins can minimize the hazardous impact of early-stage drugs.
However, the big question is whether digital twins are ready to shoulder these responsibilities in pharmaceutical drug discovery today.
Fact or fiction: The status of digital twins in healthcare
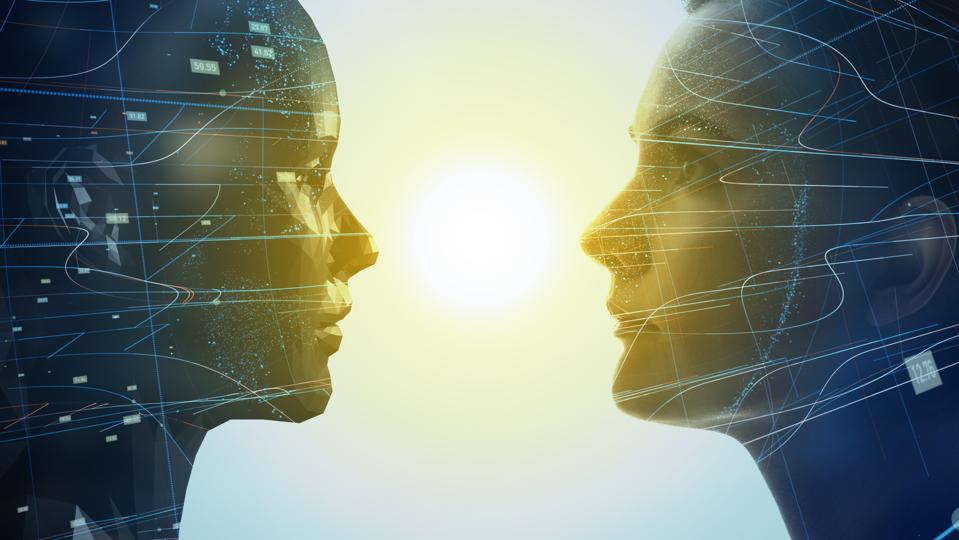
We’re still in the early days of applying digital twins to life sciences. Today, pilots use simple twins to model the molecular and cellular functions of the human body, rather than simulate the entire response of a patient in clinical trials.
“We are not at a stage yet where we can simulate the actual biochemistry of a person,” says Charles Fisher, CEO of Unlearn.AI, a startup that has raised over $17 million to build digital twins for trials. “There’s a lot of biology we don’t understand yet, and there’s no data. So, we’re not working on predicting how patients respond to brand new treatments,” he adds.
Most clinical trials split patients into two sets: the treatment arm receives the experimental drug, and the control arm receives a placebo or dummy drug. The control arm helps determine how patients with a disease respond if they do not get a new treatment.
It’s the control arm that pharmaceutical companies are first trying to transform using digital twins. “We can collect longitudinal patient data from tens of thousands of people to see how they respond to treatments. This turns the challenge into a more normal machine learning problem where you do have historical data to learn from,” Fisher explains. “We are trying to predict what will happen to a new patient receiving an existing treatment.”
In recent years, the proposed use of AI algorithms for patient care has shot up. With this trend accelerating during the pandemic, the US Food and Drug Administration (FDA) is reportedly developing regulatory frameworks for their oversight. “We are currently going through qualification procedures at FDA and European Medicines Agency (EMA) to validate that we are covered under the current guidance,” says Fisher.
What diseases could first see the use of digital twins? The potential is high for therapeutic areas where we have high-quality, high-dimensionality clinical trial and real-world data. For example, Unlearn is working on neurological disorders such as Alzheimer’s disease and multiple sclerosis.
Three roadblocks that could stall digital twins
Often, the biggest hurdles for AI innovations in going mainstream have nothing to do with technology—as demonstrated by breakthrough progress in algorithms and model accuracy. However, data collection, user adoption, and trustworthy applications haven’t caught up.
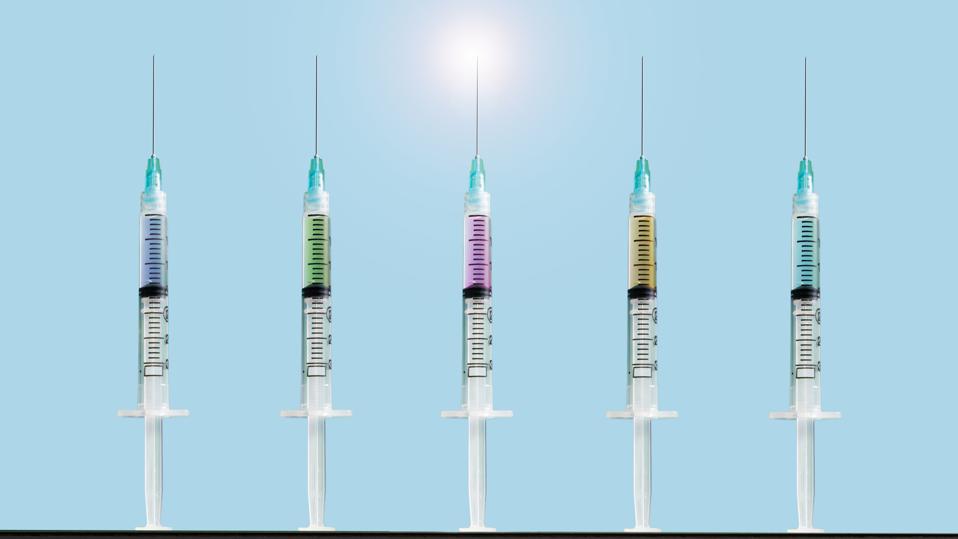
1. Healthcare’s challenges with small-scale, messy data
“Health is not just about your biology, but it is also made up of your genomics, social determinants, and everything else in between,” says Junaid Bajwa, Chief Medical Scientist at Microsoft and a practicing physician in the UK’s National Health Service (NHS). We need access to more data around these parameters to make the twins more personalized,” he adds.
While tapping into newer sources of patient data, we must also fix what we already have. Fisher adds, “Today, electronic health records [EHR] exist primarily to inform billing of patients and aren't geared for evolving the research.” He declares that the next big milestones in digital twins won’t be in AI research but from fixing the problems with small-scale, messy data in healthcare.
There is hope in sight. Digital data streams from devices such as wearables and mobile phones can enable access to high-fidelity, real-world data. Companies such as 23andMe and Datavant are working to improve the availability of high-quality healthcare data for research.
2. It’s not easy to change mindsets and behaviors
If innovators manage to build an ideal digital twin that perfectly simulates a patient’s behavior, would we jump at its adoption? The historical adoption of technological innovations suggests otherwise.
Bajwa notes, “In my clinical practice if I were asked to run diagnostics on digital twins for a day and then repeat the same process the next day on the real-life person, I would find it very odd.” It may be easy to change technology and processes but not one’s mindset.
“It took a pandemic for people to use video conferencing for online consultations,” quips Bajwa. “The technology has been around for over ten years, and its potential to enable access and equity was obvious. Yet, this low-risk change took us so much time and a global crisis.”
3. AI’s thorny issues of trust, privacy, and bias
We’ve discussed beneficial uses of this technology, but we can’t shy away from its potentially harmful consequences. “While we need high-resolution data to put digital twins to meaningful use, we must balance this effectiveness against the issues of trust, privacy, and bias,” adds Shwen Gwee, Vice President and Head of Digital Strategy at Bristol Myers Squibb.
Can patients be assured that their personalized digital twins won’t be tested on drugs or scenarios they don’t approve of? Additionally, clinical trial datasets today don’t have the best representation from an ethnicity and racial perspective. Using such biased data for training digital twins could lead to inaccurate representations of specific segments of the population.
“I don’t think you can create unbiased AI algorithms,” declares Fisher. “Since the AI is data-driven, it will reflect the bias in the data. Rather than trying to make algorithms perfect, we must focus on how we use the algorithm’s predictions in clinical trials.” Here again, the solutions don’t hinge on technology but on the right processes, human care, and involvement.
A fantastic future with AI-driven digital twins
While digital twins could kick-start a revolution by powering the synthetic control arm of clinical trials, they hold greater potential for the life sciences. Actively addressing the three challenges of data collection, user adoption, and trustworthy application could help them go mainstream.
How might a distant future with digital twins look?
“I see the potential to reduce the size of clinical trials safely and reliably, say by 25%,” says Fisher. “This can have a multiplier effect on medical research and patients. It will enable every Biotech and Pharma company to run clinical trials faster and less expensively.”
Drawing from personal experience, Bajwa shares the pain that cancer patients go through. “Though several treatment options are available today, such as surgery, chemotherapy, or immunotherapy, finding the best medicine for a patient is still a shot in the dark.”
Bajwa envisions how digital twins could transform this treatment in the future. “Let’s say my multidisciplinary clinical team is presented with a cancer patient—a 50-year-old mother of two from a suburb in London. Could we run all possible combinations on a digital twin of the patient to figure out a tailor-made treatment protocol? Doing this quickly and accurately could give the best quality of life to the individual. This can help millions survive cancer.”
That would be a fantastic future with digital twins, he concludes.
——
To watch my video interview with Charles Fisher, CEO of Unlearn AI on the future of digital twins in drug discovery, click here.