Could cameras make inspection safer and more efficient?
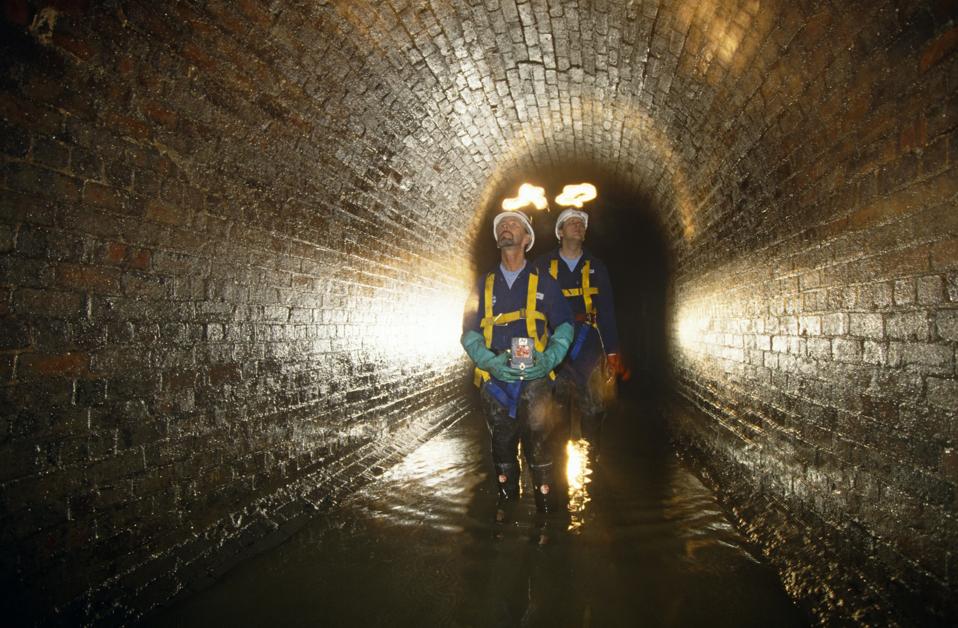
Roads, pavements, railway lines, sewer systems, water mains, electricity supplies, internet cables…. The modern world relies on a lot of physical infrastructure. Keeping each of these critical systems operating reliably doesn’t happen by magic – frequent maintenance checks and regular inspections are required. But what might surprise you to know is just how subjective infrastructure inspection has been for much of the past century. Depending on the specific system, the process can be rather... analogue. Human inspectors walk or drive along a route, manually noting the location of issues – often with pen and paper – returning soon to repair anything urgent, and scheduling a repeat visit for everything else. However skilled those inspectors may be, they’re being asked to carry out a virtually impossible and never-ending task. That’s why in recent years, a whole range of different technological tools have been developed to aid in infrastructure inspection.
Some of them, such as autonomous inspection robots that can travel through sewers and culverts, are designed to replace the human entirely. “These structures are far more hazardous to enter than a rail tunnel – they're partially flooded, and likely contain noxious gases,” says Dr Nick McCormick from the UK’s National Physical Laboratory (NPL). “Robotic platforms get people out of those dangerous situations.” Others, like a growing number of commercially available wireless sensors do something that even the best inspectors could never do. Embedded within a structure, they continuously monitor its structural health from the inside out. But many of the fastest growing technologies – particularly those based on imaging – are those that supplement the existing inspection process, making it safer, easier, and more objective.
In this article, I thought I’d introduce you to a few interesting image-based inspection projects that I’ve come across recently. Starting with one that involves the Victorian tunnels that pepper the UK rail network.
I used to be in the same NPL research division as the aforementioned McCormick (though our work was on different topics), and I interviewed him in 2015 for my book Science in the City. Back then, he and his team were working on a rail tunnel inspection system called DIFCAM – digital imaging for condition asset management. It consisted of a laser scanner combined with 11 digital SLR cameras, arranged in an arc mounted on the back of a road-rail vehicle. As the vehicle moved slowly through the tunnel, it took simultaneous images and laser scans, to build up an ultra-high-resolution image map of the tunnel wall, capable of picking up even the smallest of defects. The idea was that, by comparing maps from different inspections (using a technique called digital image correlation), they could track how (of if) those defects changed over time. With the information in hand, tunnel inspectors could then use their knowledge and judgement to make a decision on how to rectify the damage.
Though successfully tested across the National Rail network, there were two major limitations to DIFCAM – speed and positioning. “Something we wanted to experiment with was, can we locate ourselves more accurately?” says McCormick. In the old version of DIFCAM, they used a rotary odometer which measures distance by knowing the circumference of a wheel. In the new version, known as DIFCAM Evolution, they’re using a pair of event cameras from a company called Machines With Vision that point down at the ballast between the rails. McCormick explains, “These are special digital cameras that send out data from an individual pixel when there is a change of grey level in that pixel….it is effectively a real-time odometry system.” In addition, they’re using a line scan camera system that had originally been designed for doing measurement of electrical circuit boards on production lines. “The huge advantage is that the height [measurements] and images are in perfect synchronization.” The combination, he says, means they “should be able to get many 10s of meters, maybe 100 meters per second measurement speed.” This equates to measuring at 40 miles an hour, which is considered line speed on certain parts of the UK rail network. As well as removing the need to close the line for inspections, causing disruptions, it’s also ten times faster than the previous version of DIFCAM. There’s still plenty of work to be done to optimize the system, but McCormick says that the next stage will likely be a regional testing rollout. His hope is that with further funding, the system will eventually become a commercial offering.
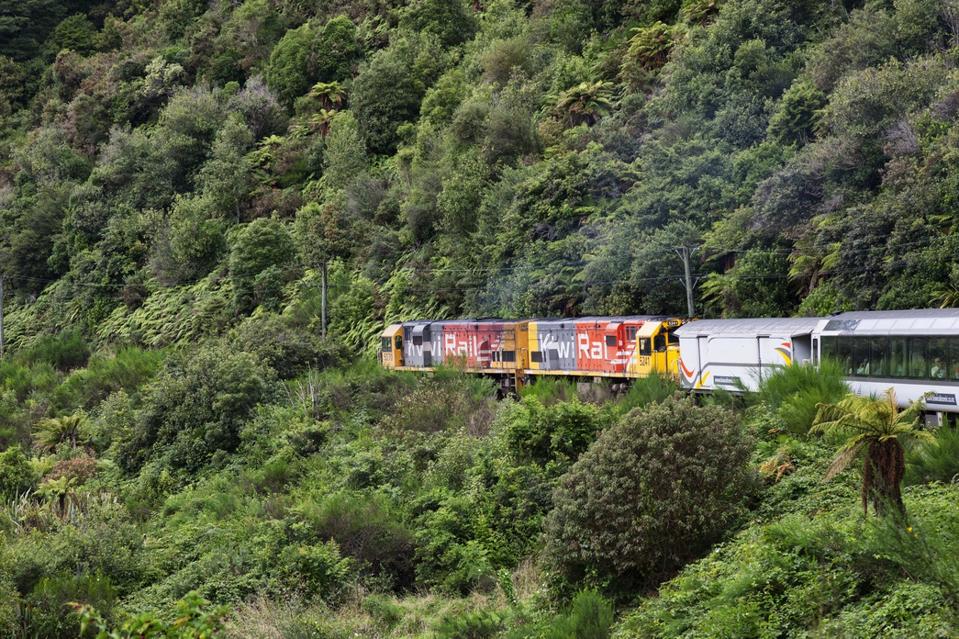
Rail is also the focus of this next project (what can I say, I love trains), but this one is based here in Aotearoa New Zealand. LIDAR, which stands for LIght Detection And Ranging, has been widely used in a whole slew of different infrastructure-related applications for several years. Possibly the most famous use is in autonomous vehicles, where LIDAR units on their roof rapidly scan and create a 3D map of the surrounding area.** Back in 2014-16, researchers at NZ’s WSP (then called Opus Research) carried out a study in which an ‘instrumented bicycle’, complete with LIDAR, was ridden on both urban and rural roads in Wellington. The goal was to measure the passing distances and speeds of motorists overtaking cyclists, and match that up with cyclists’ perceived safety. Fast-forward to late 2020, and the same system came up in a conversation between Mike Lusby, an instrumentation engineer at WSP, and Manjot Singh, Wellington infrastructure manager at KiwiRail.
“We went to visit the lab to talk about slope monitoring and some other urgent issues we were facing on the network,” says Singh. “We saw objects being left on or too close to the track after major work, which can cause big problems for trains – they could be derailed.” Lusby suggested that a LIDAR unit, supported by similar bespoke software used in the bike project, could detect obstructions on or near the line. The pair set to work, and within a few months, they’d installed LIDAR onto the front bumper of one of Kiwirail’s existing road-rail vehicle. “Hi-rail trucks are a standard piece of equipment for us. We use them to inspect our track at low speeds,” says Singh. But he admits that when it came to obstructions, inspections had previously been very subjective, “There was a human error element to it. One person would say that this object's too close, but the other person would say ‘it’s far enough away. It shouldn’t be a problem.’ It was even more challenging at night-time when visibility was limited.”
The LIDAR system did away with all that. Rather than rely on a person’s judgment, it constantly scans the tracks ahead and alerts the inspector, as Lusby explains, “In the driver’s cab, there's a button which can be green, orange, or red. Green means nothing is being detected. Orange means that something is getting closer to the rail corridor and may need to be checked in future. And then, if the system detects something inside the defined profile, it lights up red as an indication to the driver that they need to take action.”
Since being rolled out in December 2021, the system has proven to be popular with the track inspectors. For Singh, it has come with multiple benefits, “Safety is number one, of course, but next are the economics. In the past we’ve had quite significant damage to some of the trains, up to a hundred thousand dollars, because of things being left too close to the track. Plus there’s the disruption itself and the damage to our reputation. [This project] has been a pretty big deal for us.”
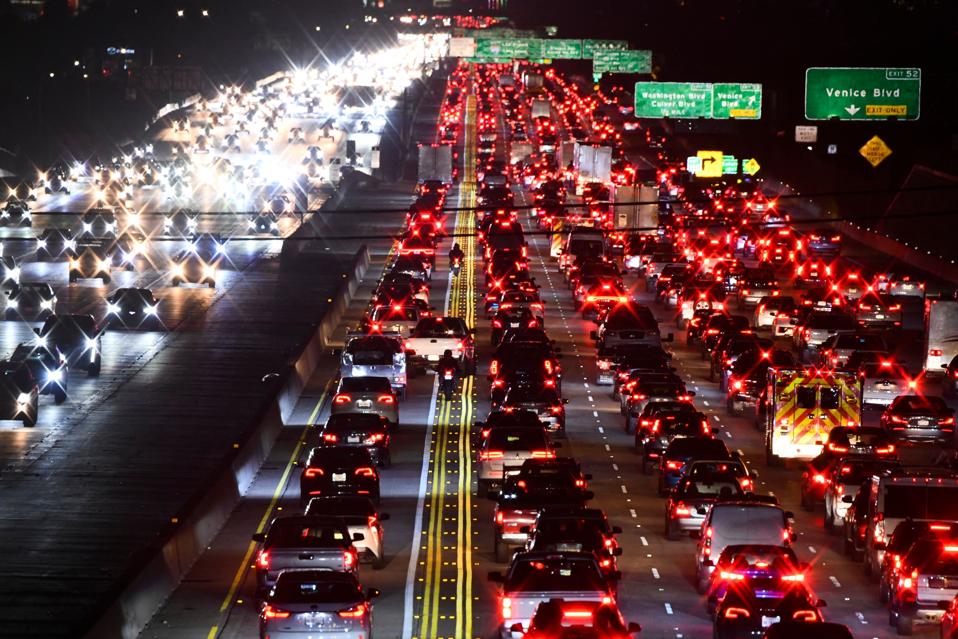
Swaroop Patnaik started his career in medical imaging, but these days, he’s focused on infrastructure inspection. His start-up company, Blue Dome Technologies can extract useful information such as the condition of a road surface, the location of streetlights, or the night-time reflectivity of signs, all from video footage. Rather than use a purpose-built unit to gather the information, all that’s needed is a basic GoPro camera mounted onto the front of a vehicle, and for that vehicle to be driven on the route of interest at normal road speeds. “You can buy a GoPro for less than $500,” says Patnaik, speaking to me from California. “Compare that to some of the really sophisticated instrumented trucks that many transportation departments use – they can cost anywhere between $400,000 and $1,000,000.”
This video footage is then uploaded to BlueDome’s machine learning platform, where, depending on the requirement, it is passed through a series of different algorithm packages. “If the customer is interested in signs, we pass the video through some AI that has been trained to identify these signs,” he explains. “But from that same video we can identify manhole covers, sewer inlets, fire hydrants, street lights – all the assets that a city or county might be interested in. We’ve purposely built the AI as separate units so people just get the information they need.”
One of the uses I was most interested in was its ability to analyze road condition. Anyone who has driven in the US will know that a lot of interstate roads are built with concrete slabs as the top surface. Checking the condition of these slabs is usually done manually, by multiple inspectors sitting in a vehicle, or by closing sections of the road and walking along it. Patnaik showed me a GoPro video captured by the California Department of Transportation (Caltrans) at highway speed that had been passed through his machine learning platform. In it, flashes of purple continuously appeared, highlighting the location of cracks in the road, and noting the area, width and length of those cracks. “All of that is automatically calculated based on the industry standard in the US.” he explains. “We identify these cracks and then we tell them which slab number it is on. It means that Caltrans know the location and dimensions of the crack they need to fix before they go out.”
The long term goal is to use all of this data to predict when a particular asset needs to be replaced, as Patnaik explains “Our thinking was that if data capture is easy, then we can get a lot of data, and ultimately might be able to predict prior to something happening. Let's say, the deterioration of a road sign. If we can collect this data over time, we’ll get an idea of how quickly that type of sign deteriorates. It might allow the city or county to be little more proactive, and to plan maintenance and repairs ahead of time.” To date, Patnaik’s work has focused on California, but his hope is that it will be rolled out more widely, “It should be able to be used everywhere. The idea is simple. If the human eye can see it, the GoPro can capture it, and we can train the AI to analyze it.”
** LIDAR is not used in Tesla’s ‘self-driving’ cars. They’ve adopted a computer vision system based on cameras. Some argue that this is more limited (and less safe) than LIDAR.