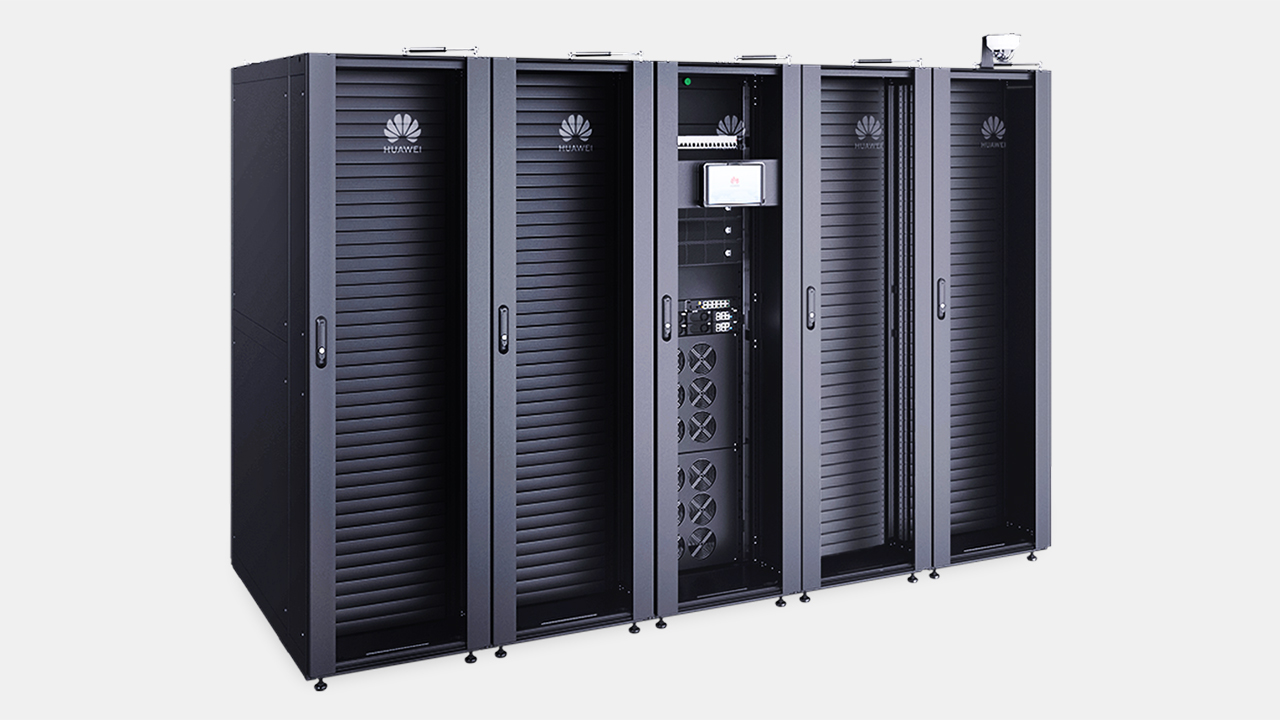
Unable to use leading-edge process technologies to produce its high-end processors for AI, Huawei has to rely on brute force – install more processors than its industry competitors to achieve comparable performance for AI.
To do this, Huawei took a multifaceted strategy that includes a dual-chiplet HiSilicon Ascend 910C processor, optical interconnections, and the Huawei AI CloudMatrix 384 rack-scale solution that relies on proprietary software, reports SemiAnalysis. The whole system provides a 2.3X lower performance per watt than Nvidia's GB200 NVL72, but it still enables Chinese companies to train advanced AI models.
At glance
Huawei's CloudMatrix 384 is a rack-scale AI system composed of 384 Ascend 910C processors arranged in a fully optical, all-to-all mesh network. The system spans 16 racks, including 12 compute racks housing 32 accelerators each and four networking racks facilitating high-bandwidth interconnects using 6,912 800G LPO optical transceivers.
Unlike traditional systems that use copper wires for interconnections, CloudMatrix relies entirely on optics for both intra- and inter-rack connectivity, enabling extremely high aggregate communication bandwidth. The CloudMatrix 384 is an enterprise-grade machine that features fault-tolerant capabilities and is designed for scalability.
In terms of performance, the CloudMatrix 384 delivers approximately 300 PFLOPs of dense BF16 compute, which is nearly two times the throughput of Nvidia’s GB200 NVL72 system (which delivers about 180 BF16 PFLOPs). It also offers 2.1 times more total memory bandwidth despite using HBM2E and over 3.6 times greater HBM capacity. The machine also features 2.1 times higher scale-up bandwidth and 5.3 times scale-out bandwidth thanks to its optical interconnections.
However, these performance advantages come with a tradeoff: The system is 2.3 times less power-efficient per FLOP, 1.8 times less efficient per TB/s of memory bandwidth, and 1.1 times less efficient per TB of HBM memory compared to Nvidia.
Comparison between Nvidia's GB200 NVL72 and Huawei's CloudMatrix CM384
But this does not really matter, as Chinese companies (including Huawei) cannot access Nvidia's GB200 NVL72 anyway. So if they want to get truly high performance for AI training, they will be more than willing to invest in Huawei's CloudMatrix 384.
At the end of the day, the average electricity price in mainland China has declined from $90.70 MWh in 2022 to $56 MWh in some regions in 2025, so users of Huawei's CM384 aren't likely to go bankrupt because of power costs. So, for China, where the energy is abundant, but advanced silicon is constrained, Huawei's approach to AI seems to work just fine.
HiSilicon Ascend 910C: Huawei goes dual-chiplet
When we first encountered Huawei's HiSilicon Ascend 910C processor several months ago, it was a die shot of its compute chiplet, presumably produced by SMIC, which had an I/O that was supposed to connect it to its I/O die. This is why we thought it was a processor with one compute chiplet. We were wrong.
Apparently, the HiSilicon Ascend 910C is a dual-chiplet processor with eight HBM2E memory modules and without an I/O die that resembles AMD's Instinct MI250X and Nvidia's B200. The unit delivers 780 BF16 TFLOPS compared to MI250X's 383 BF16 TFLOPS and B200's 2.25 - 2.5 BF16 TFLOPS.
Comparison between Nvidia's B200 and Huawei's Ascend 910C
The HiSilicon Ascend 910C was designed in China for large-scale training and inference workloads. The processor is was designed using advanced EDA tools from well-known companies and can be produced using 7nm-class process technologies. SemiAnalysis reports that while SMIC can produce compute chiplets for the Ascend 910C, the vast majority of Ascend 910C chiplets used by Huawei were made by TSMC using workarounds involving third-party entities like Sophgo, allowing Huawei to obtain wafers despite U.S. restrictions. It is estimated that Huawei acquired enough wafers for over a million Ascend 910C processors from 2023 to 2025. Nonetheless, as SMIC's capabilities improve, Huawei can outsource more production to the domestic foundry.
The Ascend 910C uses HBM2E memory, most of which is sourced from Samsung using another proxy, CoAsia Electronics. CoAsia shipped HBM2E components to Faraday Technology, a design services firm, which then worked with SPIL to assemble HBM2E stacks alongside low-performance 16nm logic dies. These assemblies technically complied with U.S. export controls because they did not exceed any thresholds outlined by the U.S. regulations. The system-in-package (SiP) units were shipped to China only to have their HBM2E stacks desoldered to be shipped to Huawei, which then reinstalled them on its Ascend 910C SiPs.
In performance terms, the Ascend 910C is considerably less powerful on a per-chip basis than Nvidia's latest B200AI GPUs, but Huawei's system design strategy compensates for this by scaling up the number of chips per system.
More processors = more performance
Indeed, as the name suggests, the CloudMatrix 384 is a high-density computing cluster composed of 384 Ascend 910C AI processors, physically organized into a 16-rack system with 32 AI accelerators per rack. Within this layout, 12 racks house compute modules, while four additional racks are allocated for communication switching. Just like with Nvidia's architecture, all Ascend 910Cs can communicate with each other as they are interconnected using a custom mesh network.
However, a defining feature of the CM384 is its exclusive reliance on optical links for all internal communication within and between racks. It incorporates 6,912 linear pluggable optical (LPO) transceivers, each rated at 800 Gbps, resulting in a total internal bandwidth exceeding 5.5 Pbps (687.5 TB/s) at low latency and with minimal signal integrity losses. The system supports both scale-up and scale-out topologies: scale-up via the full-mesh within the 384 processors, and scale-out through additional inter-cluster connections, which enables deployment in larger hyperscale environments while retaining tight compute integration.
With 384 processors, Huawei's CloudMatrix 384 delivers 300 PFLOPs of dense BF16 compute performance, which is 166% higher compared to Nvidia's GB200 NVL72. However, all system power (including networking and storage) of the CM384 is around 559 kW, whereas Nvidia's GB200 NVL72 consumes 145 kW.
As a result, Nvidia's solution delivers 2.3 times higher power efficiency than Huawei's solution. Still, as noted above, if Huawei can deliver its CloudMatrix 384 in volumes, with proper software and support, the last thing its customers will care about is the power consumption of their systems.