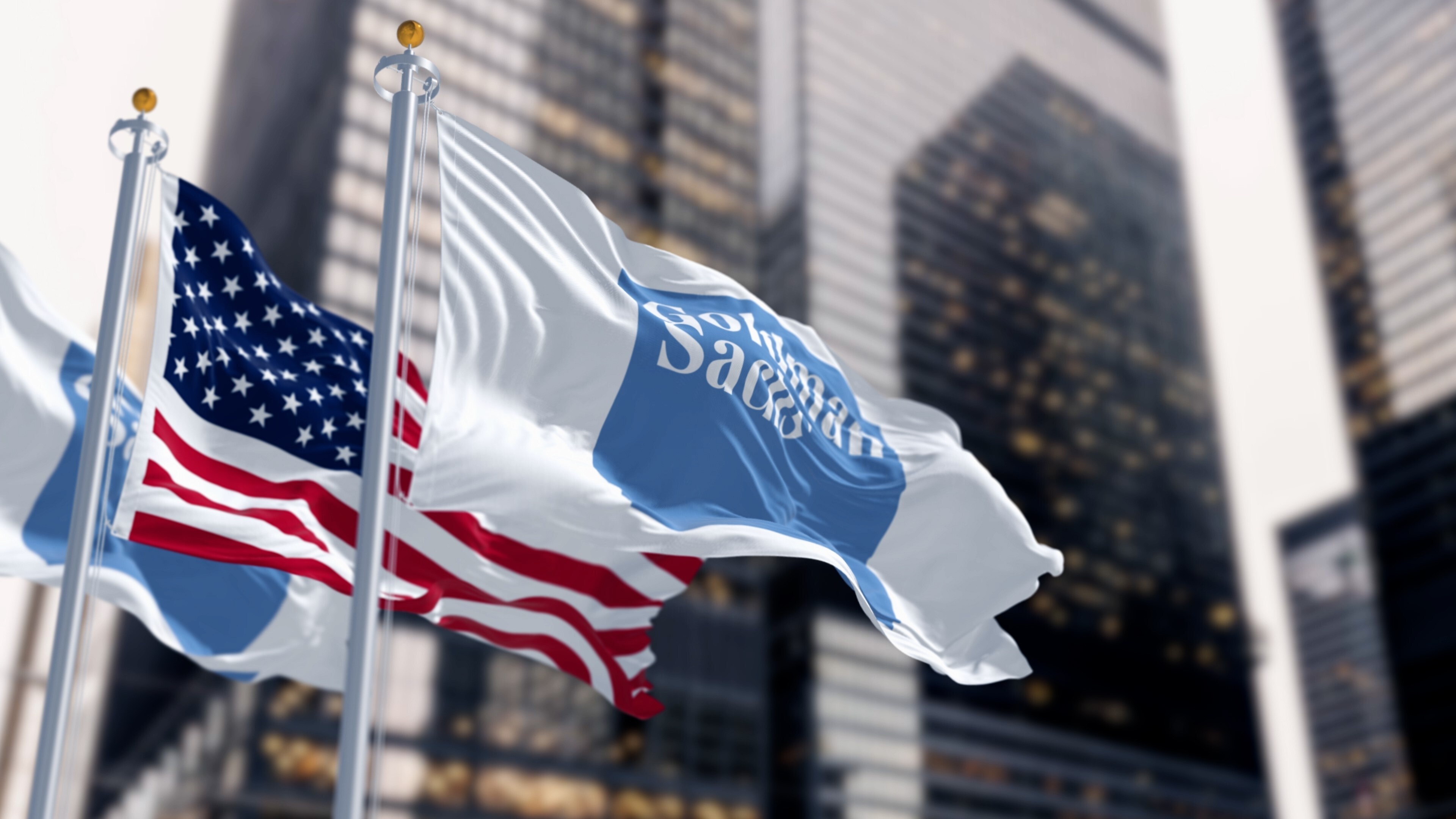
Corporations and investors have been spending billions of dollars on building AI. The current LLM models we use today, like GPT-4o, already cost hundreds of millions of dollars to train, and the next-generation models are already underway, going up to a billion dollars. However, Goldman Sachs, one of the leading global financial institutions, is asking whether these investments will ever pay off.
Sequoia Capital, a venture capital firm, recently examined AI investments and computed that the entire industry needs to make $600 billion annually just to break even on its initial expenditure. So, as massive corporations like Nvidia, Microsoft, and Amazon are spending huge amounts of money to gain a leg up in the AI race, Goldman Sachs interviewed several experts to ask whether investments in AI will actually pay off.
The expert opinions in the Goldman Sachs report are currently divided into two groups: one group is skeptical about its group, saying that AI will only deliver limited returns to the American economy and that it won’t solve complex problems more economically than current technologies. On the other hand, the opposing view says that the capital expenditure cycle on AI technologies seems promising and is similar to what prior technologies went through.
MIT Professor Daron Acemoglu estimates that generative AI’s impact on the economy will be limited, contributing only to around a 0.5% increase in productivity and a 1% addition to GDP output. This sharply contrasts estimates by Goldman Sachs’s economists, who suggested a 9% jump in productivity and a 6.1% increase in GDP. He also said that even though AI technologies will eventually evolve and become less costly, he isn’t convinced that the current trend of dumping more data and computing power at AI models will allow us to hit our vision of artificial general intelligence more quickly.
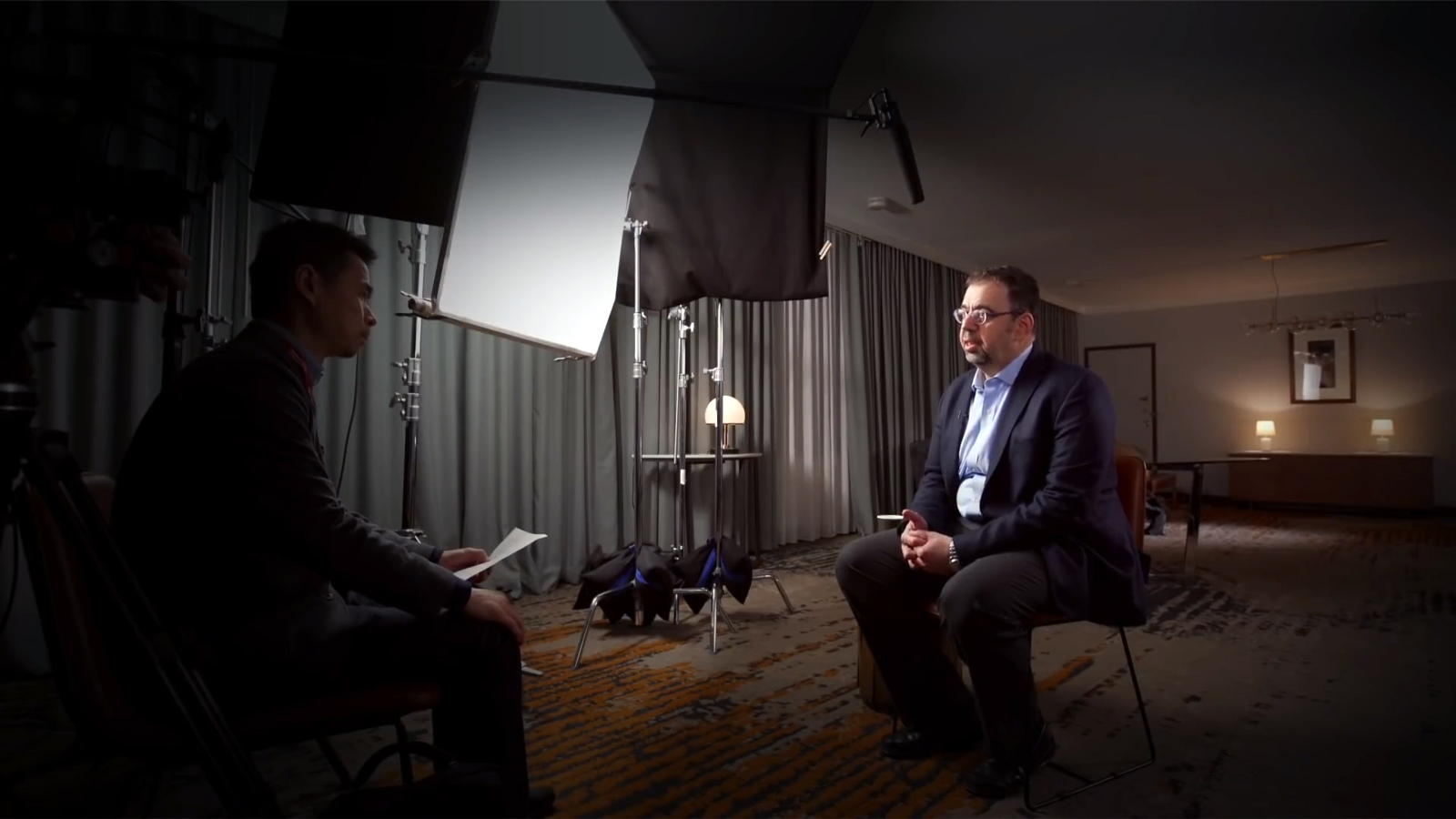
“Human cognition involves many types of cognitive processes, sensory inputs, and reasoning capabilities. Large language models (LLMs) today have proven more impressive than many people would have predicted, but a big leap of faith is still required to believe that the architecture of predicting the next word in a sentence will achieve capabilities as smart as HAL 9000 in 2001: A Space Odyssey,” said Acemoglu. “It’s all but certain that current AI models won’t achieve anything close to such a feat within the next ten years.”
The contrarian view on the report comes from Kash Rangan and Eric Sheridan, both Senior Equity Research Analysts at Goldman Sachs. They say that even though returns on AI investments are taking longer than expected, they should eventually pay off. Rangan says, “Every computing cycle follows a progression known as IPA — infrastructure first, platforms next, and applications last. The AI cycle is still in the infrastructure buildout phase, so finding the killer application will take more time, but I believe we’ll get there.”
“This capex (capital expenditure) cycle seems more promising than even previous capex cycles because incumbents — rather than upstarts — are leading it, which lowers the risk that technology doesn’t become mainstream,” Sheridan added. “Incumbents [like Microsoft and Google] have access to deep pools of capital, an extremely low cost of capital, and massive distribution networks and customer bases, which allows them to experiment with how the capital dollars could eventually earn a return.”
Despite these two contrarian views, Goldman Sachs recognized AI’s two challenges—the availability of chips and power. The AI GPU crunch seems to be over, primarily because Nvidia can now deliver chips with a lead time of two to three months instead of the 11 months it used to take.
However, data center power consumption is now the primary limiting factor, especially as AI GPUs are increasingly power-hungry. A single modern AI GPU could use up to 3.7 MWh of power annually, with all the GPUs sold just last year consuming enough electricity to power more than 1.3 million average American households. Major corporations have even now started looking at modular nuclear power plants just to ensure that their massive AI data centers can get the power they require.
Only history can tell us whether AI will boom like the internet and e-commerce or bust like 3D TVs, virtual reality, and the metaverse. But whatever the case, we expect to see AI development continue. Goldman Sachs says, “We still see room for the AI theme to run, either because AI starts to deliver on its promise, or because bubble take a long time to burst.”