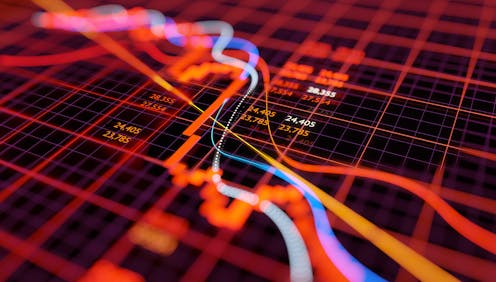
According to the infamous myth, groups of lemmings sometimes run off cliffs to their collective doom. Imagine you are one of these rodents: on a sunny day you join your companions in a joyous climb up a mountain beneath clear skies, traipsing across grass and dirt and rock, glad to be among friends, until suddenly you plunge through the brisk air and all goes black.
The edge of the cliff is what scientists call a “critical point”: the spot where the behaviour of a system (such as a group of lemmings) suddenly goes from one type of state (happily running) to a very different type of state (plummeting), often with catastrophic results.
Lemmings don’t actually charge off cliffs, but many real-world systems do experience critical points and abrupt disasters, such as stock market crashes, power grid failures, and tipping points in climate systems and ecosystems.
Critical points aren’t always literal points in space or time. They can be values of some system parameter – such as investor confidence, environmental temperature, or power demand – that marks the transition to instability.
Can we tell when a system is close to a cliff, and perhaps act to stop it going off the edge? What can we measure about a share market or ecosystem that could help us predict how far it is from such a critical point?
We have developed a new method for doing exactly this in real-world systems. Our work is published this week in Physical Review X.
How do you know when you’re close to a cliff?
Previous work has shown that systems tend to “slow down” and become more variable near critical points. For a share market, for example, this would mean stock prices changing less rapidly and exhibiting a larger difference between weekly highs and lows.
But these indicators don’t work when systems are “noisy”, meaning we can’t measure what they are doing very accurately. Many real systems are very noisy.
Are there indicators that do work for real-world systems? To find out, we searched through more than 7,000 different methods in hope of finding one powerful enough to work well, even when there is lots of noise in our system.
We found a few needles in our haystack: a handful of methods that performed surprisingly well at this very difficult problem. Based on these methods, we formulated a simple new recipe for predicting critical points.
We gave it an appropriately awesome name: RAD. (This gnarly acronym has a very nerdy origin: an abbreviation of “Rescaled AutoDensity”.)
Do brains use critical points for good?
We verified our new method on incredibly intricate recordings of brain activity from mice. To be more specific, we looked at activity in areas of the mouse brain responsible for interpreting what the mouse sees.
When a neuron fires, neighbouring neurons might pick up its signal and pass it on, or they might let it die away. When a signal is amplified by neighbours it has more impact, but too much amplification and it can cross the critical point into runaway feedback – which may cause a seizure.
Our RAD method revealed that brain activity in some regions has stronger signs of being close to a critical point than others. Specifically, areas with the simplest functions (such as size and orientation of objects in an image) work further from a critical point than areas with more complex functions.
This suggests the brain may have evolved to use critical points to support its remarkable computational abilities.
It makes sense that being very far from a critical point (think of safe lemmings, far from the cliff face) would make neural activity very stable. Stability would support efficient, reliable processing of basic visual features.
But our results also suggest there’s an advantage to sitting right up close to the cliff face – on the precipice of a critical point. Brain regions in this state may have a longer “memory” to support more complex computations, like those required to understand the overall meaning of an image.
A better guide to cliffs
This idea of systems sitting near to, or far from, a critical point, turns up in many important applications, from finance to medicine. Our work introduces a better way of understanding such systems, and detecting when they might exhibit sudden (and often catastrophic) changes.
This could be used to unlock all sorts of future breakthroughs – from warning individuals with epilepsy of upcoming seizures, to helping predict an impending financial crash.

Ben Fulcher receives funding from the Australian Research Council and National Health and Medical Research Council.
Brendan Harris does not work for, consult, own shares in or receive funding from any company or organisation that would benefit from this article, and has disclosed no relevant affiliations beyond their academic appointment.
This article was originally published on The Conversation. Read the original article.