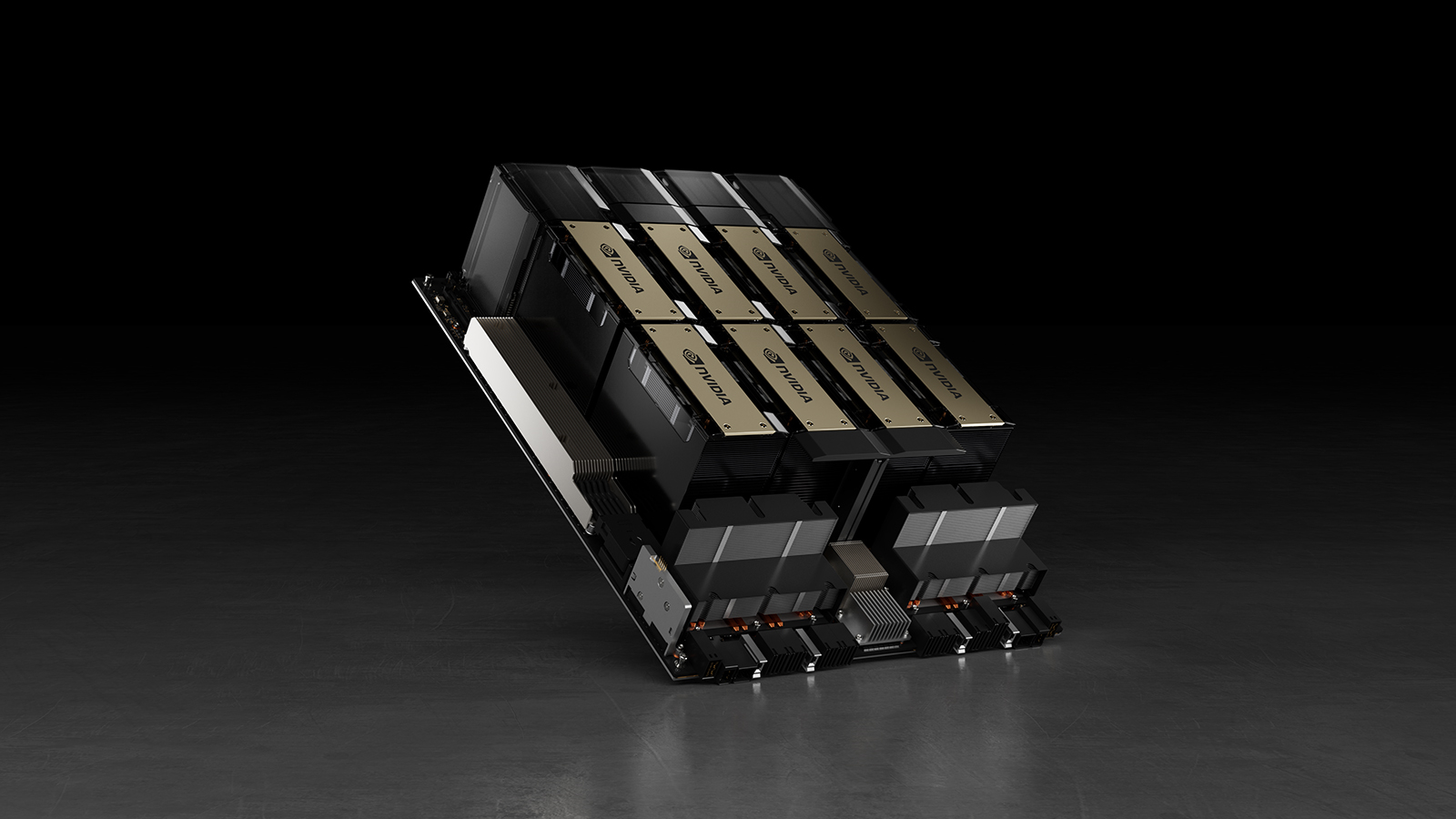
As Chinese entities do not have access to tens of thousands of advanced AI GPUs from companies like Nvidia, companies from this country must innovate to train their advanced AI models. Kai-Fu Lee, the founder and head of 01.ai, said this week that his company has trained one of its advanced AI models using 2,000 GPUs with just $3 million.
“The thing that shocks my friends in the Silicon Valley is not just our performance, but that we trained the model with only $3 million and GPT-4 was trained with $80 to $100 million,” said Kai-Fu Lee (via @tsarnick). “GPT-5 is rumored to be trained with about a billion dollars. […] We believe in scaling law, but when you do excellent detailed engineering, it is not the case. […] As a company in China, first, we have limited access to GPUs due to the U.S. regulations [and a valuation disadvantage compared to American AI companies].”
In contrast to competitors like OpenAI, which spent $80-100 million to train GPT-4 and reportedly up to $1 billion for GPT-5, 01.ai trained its high-performing model with just $3 million, according to Kai-Fu Lee. According to a company website chart, 01.ai's Yi-Lightning holds the sixth position in model performance measured by LMSIS at UC Berkeley.
This accomplishment is, of course, particularly notable because of the company’s impressive cost-efficiency. The stark difference demonstrates that achieving top-tier AI capabilities does not always require enormous budgets, as careful engineering and targeted optimizations can yield similar results at a fraction of the cost and the compute resources.
It is believed that OpenAI used 10,000 Nvidia A100 GPUs to train its GPT-3 model and many more H100 processors to train its GPT-4 and GPT-4o models. Using various innovative solutions, 01.ai had to train its Yi-Lightning model using 2,000 undisclosed GPUs. However, last year, Kai-Fu Lee said his company had enough GPUs to execute its roadmap for 1.5 years. The numbers, however, do not add up. One Nvidia H100 GPU costs around $30,000, so two thousands of such GPUs would cost $6 million.
To enhance model performance, 01.ai focused on reducing the bottlenecks in its inference process by turning computational demands into memory-oriented tasks, building a multi-layer caching system, and designing a specialized inference engine to optimize speed and resource allocation. As a result, ZeroOne.ai’s inference costs are dramatically lower than those of similar models — 10 cents per million tokens — about 1/30th of the typical rate comparable models charge.
“When we only have 2000 GPUs, the team has to figure out how to use it,” said Kai-Fu Lee. “I, as the CEO, have to figure out how to prioritize it, and then not only do we have to make training fast, but we also have to make inference fast. So, our inference is designed by figuring out the bottlenecks in the entire process by trying to turn a computational problem to a memory problem, by building a multi-layer cache, by building a specific inference engine, and so on. But the bottom line is, our inference cost is 10 cents per million tokens.”
Chinese companies, in general and 01.ai in particular, face significant challenges. Due to U.S. export restrictions, they have minimal access to advanced GPUs. They also have a valuation disadvantage compared to American AI companies, which affects their available resources and investment opportunities.