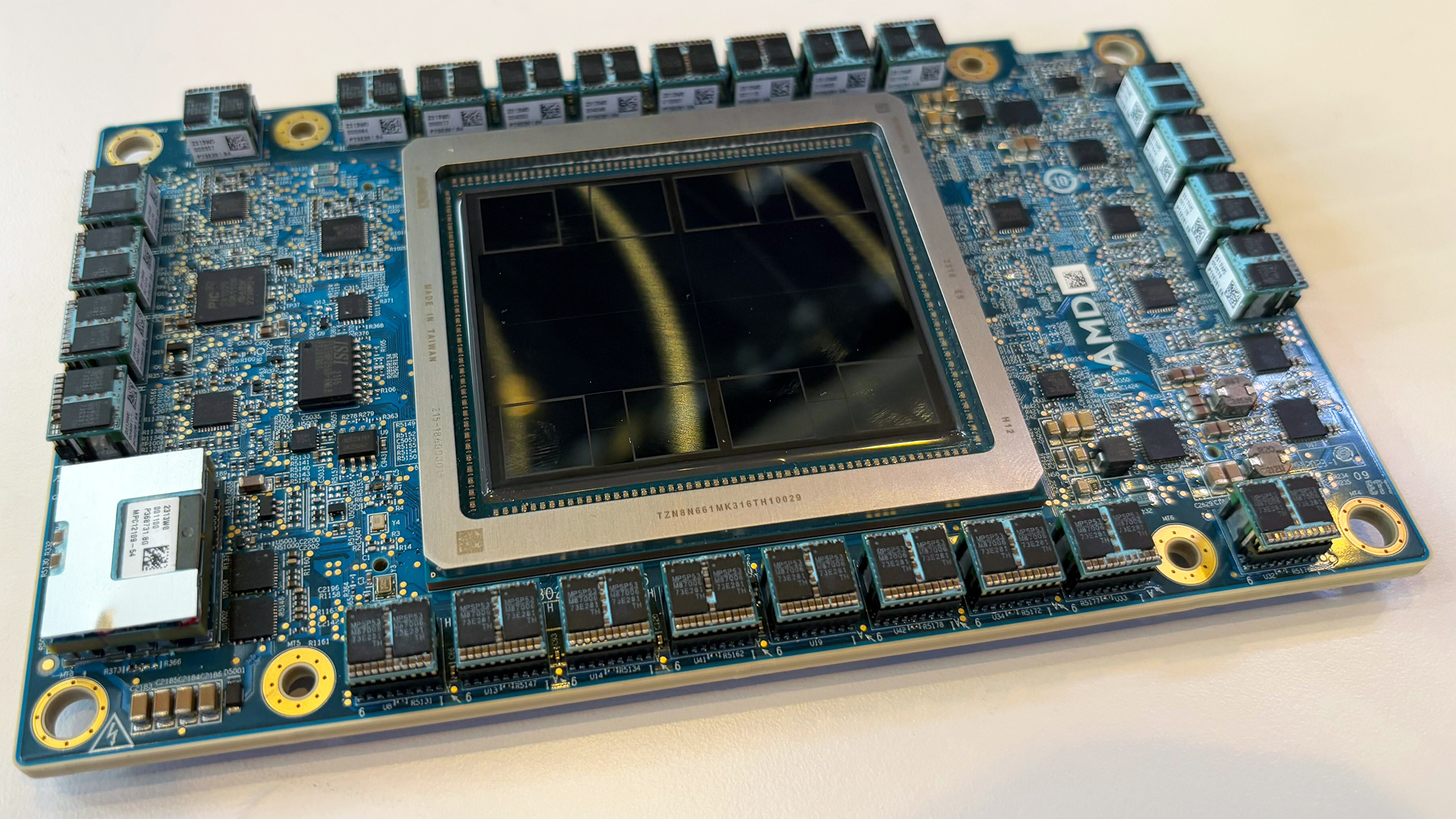
At its CES exposition, AMD demonstrated its latest Instinct MI325X accelerator for AI and HPC workloads, which also happens to be the world's only processor with 256 GB of HBM3E memory onboard and promises to be one of the most efficient GPUs for inference.
Although the Consumer Electronics Show is meant to demonstrate the latest and greatest electronics designed for consumers, semiconductor companies have long used CES to showcase technologies that they deem fit for the show. While Nvidia spent most of its keynote talking about AI, AMD actually introduced a range of processors for client PCs, but this certainly does not mean that the company had nothing to show. In fact, it demonstrated its all-new Instinct MI325X.
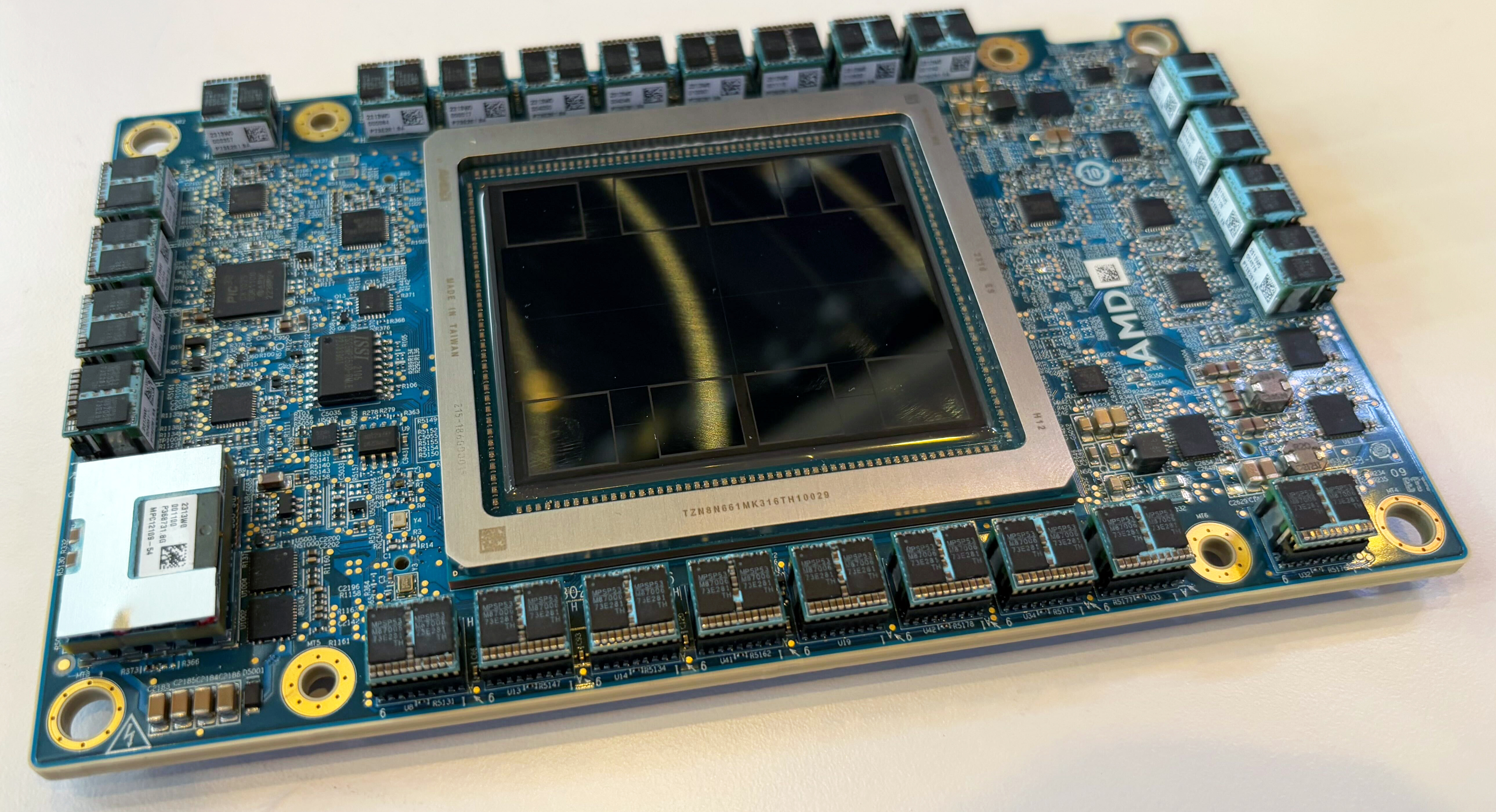
AMD's Instinct MI325X comes with the same dual-chiplet GPU that powers the Instinct MI300X and features 19,456 stream processors (304 compute units) clocked at up to 2.10 GHz. However, the new accelerator is equipped with 256 GB of HBM3E memory featuring 6 TB/s of bandwidth, as opposed to 192 GB of HBM3 memory with 5.3 TB/s of bandwidth.
As Nvidia's H200 comes with 'only' 141 GB of HBM3E memory with 4.8 TB/s of bandwidth, AMD's Instinct MI325X leads the industry in terms of HBM3E memory capacity onboard. Interestingly, AMD had previously announced that the MI325X would come with 288 GB of HBM3E, but then decided to reduce the usable capacity to 256 GB of memory for an unknown reason.
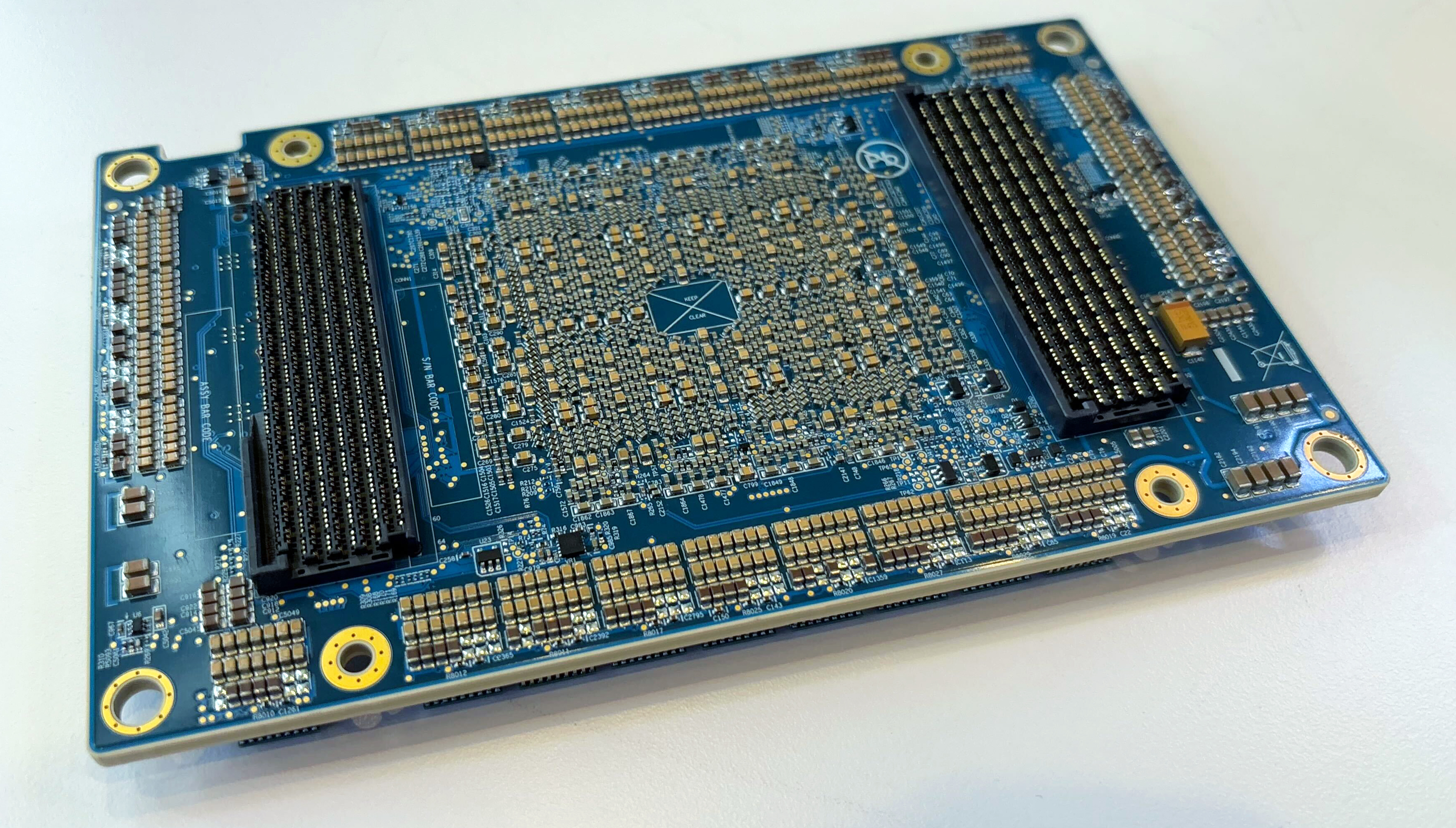
Having more onboard memory is crucial for AI accelerators, both for training and for inference, at least in theory.
Modern AI models usually have tens of billions of parameters and require tens of thousands of GPUs for training. Storing these parameters, along with intermediate data and gradients, requires a substantial amount of memory. Since no such model fits into a GPU's onboard memory, developers have to employ techniques like model parallelism or tensor slicing, which add computational and communication overhead. With more GPU memory, fewer GPUs are needed for training due to lower overheads.
Additionally, AI accelerators process data in batches. A larger onboard memory capacity allows for bigger batches, which can lead to higher throughput and faster, more efficient training and inference. Smaller memory forces the model to run with smaller batch sizes, reducing efficiency.
However, things look somewhat different in the real world. A system with eight Nvidia H100 80 GB GPUs generates a comparable number of tokens per second to a machine with eight AMD Instinct MI300X 192 GB GPUs in the MLPerf 4.1 generative AI benchmark on the Llama 2 70B model, according to data submitted by AMD and Nvidia as of late August. By contrast, an 8-way server with H200 141 GB GPUs generates over 30% more tokens per second compared to an 8-way MI300X 192 GB machine.
For now, it seems that the Instinct MI300X was unable (at least as of August) to fully utilize its hardware capabilities, likely due to limitations in the software stack. It remains to be seen whether the Instinct MI325X will overcome the same software stack limitations and succeed in outperforming its rivals.