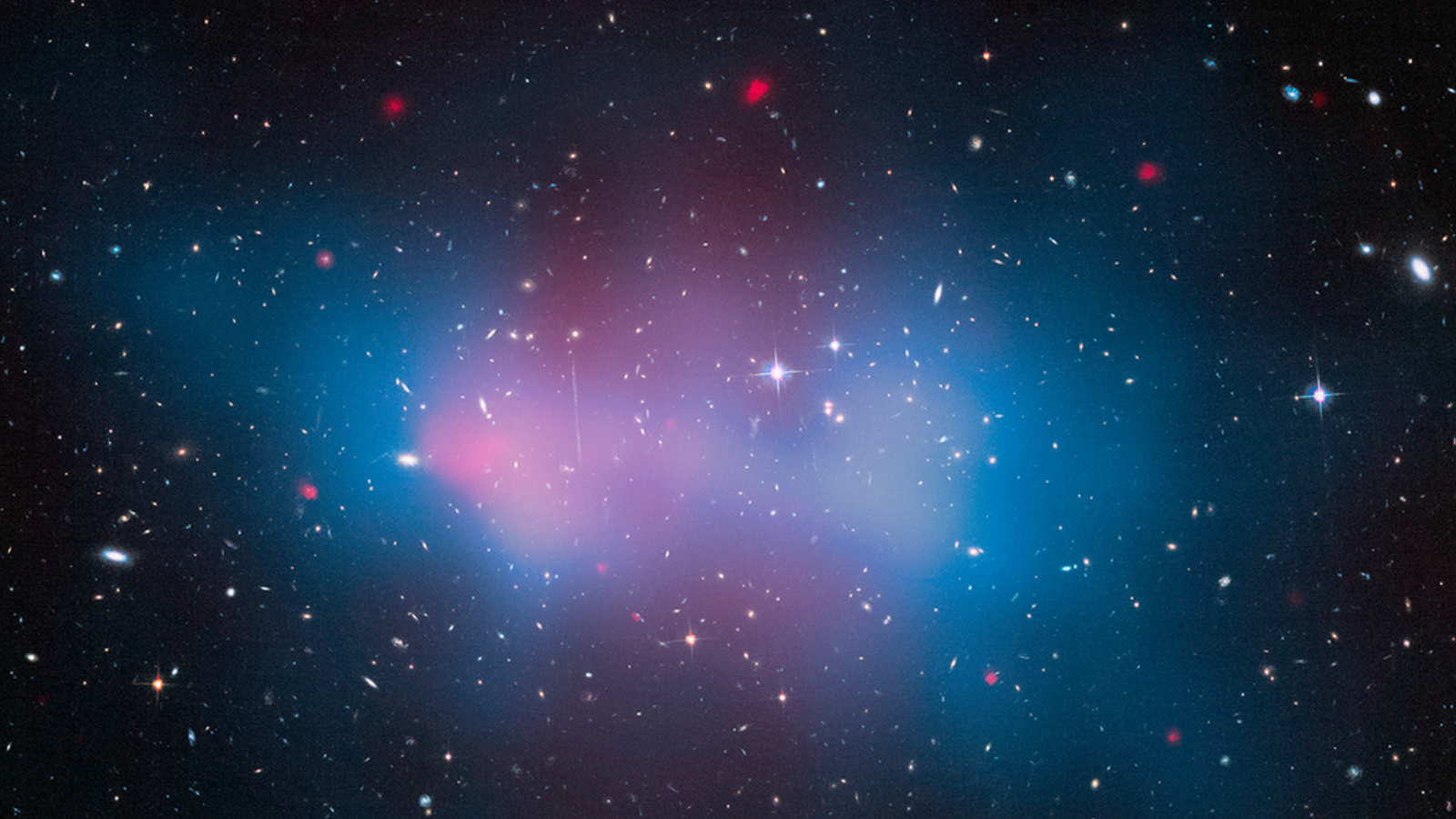
It was only a matter of time before artificial intelligence took on dark matter. A new deep-learning algorithm is set to be unleashed upon images of galaxy clusters in search of the telltale signs of this invisible substance that strangely makes up 85% of all matter in the universe.
According to the standard model of cosmology, every galaxy is surrounded by a halo of dark matter. Similarly, galaxy clusters are suffused inside vast haloes of dark matter, which we can detect indirectly. Scientists are also able to determine dark matter's distribution in a cluster by watching for the way its gravitational influence bends space, therefore creating weak, and sometimes strong, gravitational lenses. Yet, despite the huge volumes of dark matter in the universe, nobody knows what it is made from.
Occasionally, two galaxy clusters — containing galaxies, hot gas and dark matter — can collide. When this happens, how the collision proceeds depends on the nature of dark matter.
It all comes down to a property of dark matter known as its interaction cross section, which refers to the basis by which dark matter is an unidentified type of particle. One of the reasons astronomers have had so much difficulty tracking down the identity of dark matter is that it doesn't seem to interact with normal matter, other than through gravity. However, some models predict that particles of dark matter can interact with each other, and to what extent this interaction takes place depends upon the interaction cross section.
Related: Dark energy remains a mystery. Maybe AI can help crack the code
So, when two galaxy clusters collide, the fate of their dark matter haloes depends upon this cross section. If the value of the cross section is high, the particles in the two dark matter haloes that are colliding will interact, slowing the dark matter down. Galaxies, on the other hand, will sail on through, rarely actually "colliding" in the way you may think because of the large spaces within stars and other objects within them. Meanwhile, huge clouds of hydrogen in the cluster do collide, growing hot and radiating X-rays.
If the value of the interaction cross section is high, the dark matter will separate from the galaxies and get distributed closer to the hot gas clouds.
Alternatively, if dark matter has a small cross section, then the dark matter and galaxies would be separated, but not by as much, with the dark matter found between the galaxies and the hot gas. If the cross section is zero, meaning that dark matter is collisionless, then we should expect the dark matter haloes to stay with the galaxies as they would pass right through each other without interacting at all.
However, there are several complications. One is that we can see only snapshots of galaxy cluster collisions because they take place over time and distance scales that are far too large to reveal progress on human timescales. Furthermore, we are seeing these snapshots all at different stages of collisions and from different angles, so no two galaxy cluster mergers look exactly the same, and it requires a trained eye to pluck what is happening from each example.
A second complication is the effect that winds of radiation from galaxies with active black holes can have. These features are commonly found in the largest galaxies within a cluster, such as M87 in the Virgo galaxy cluster. These radiation winds, described as "feedback" because they directly affect what ultimately instigates them, specifically matter falling towards the central black hole. This feedback can push matter out of a galaxy and into the extragalactic medium within a galaxy cluster, so that ordinary matter ends up where the dark matter might be expected to reside.
To help distinguish among the possibilities, David Harvey of the Ecole Polytechnique Fédérale de Lausanne in Switzerland has written a deep-learning algorithm trained on simulated images of galaxy cluster collisions from the BAHAMAS (Baryons and Haloes of Massive Systems) project conducted by researchers from Liverpool John Moores University, Leiden University, Johns Hopkins University and CNRS in France.
The simulations model galaxy cluster collisions with different cross-sectional values, and even those with no dark matter at all.
Harvey tested different versions of his algorithm, which is a Convolutional Neural Network (CNN) able to recognize patterns in images very well. Harvey found that the most complex version of his algorithm, nicknamed "Inception," was the most accurate, scoring an 80% success rate when challenged to characterize the simulated cluster collisions.
Several projects are already imaging galaxy cluster collisions in an attempt to solve the mystery of dark matter. The Hubble Space Telescope, with assistance from the Chandra X-ray Observatory, has been imaging galaxy cluster collisions for some time now, most famously the Bullet Cluster in 2006. More recently, the European Space Agency launched the Euclid mission, which is designed to study the so-called "dark universe" including the presence of dark matter in clusters. And on a smaller scale, the high-altitude balloon mission called SuperBIT flew around the world for two months in 2023 imaging galaxy cluster collisions, before crash-landing in Argentina. With all this observational data, and more to come, Harvey's "Inception" algorithm will help us find a faster answer to the puzzle that is dark matter.
Harvey's algorithm and its results were described on Sept. 6 in Nature Astronomy.