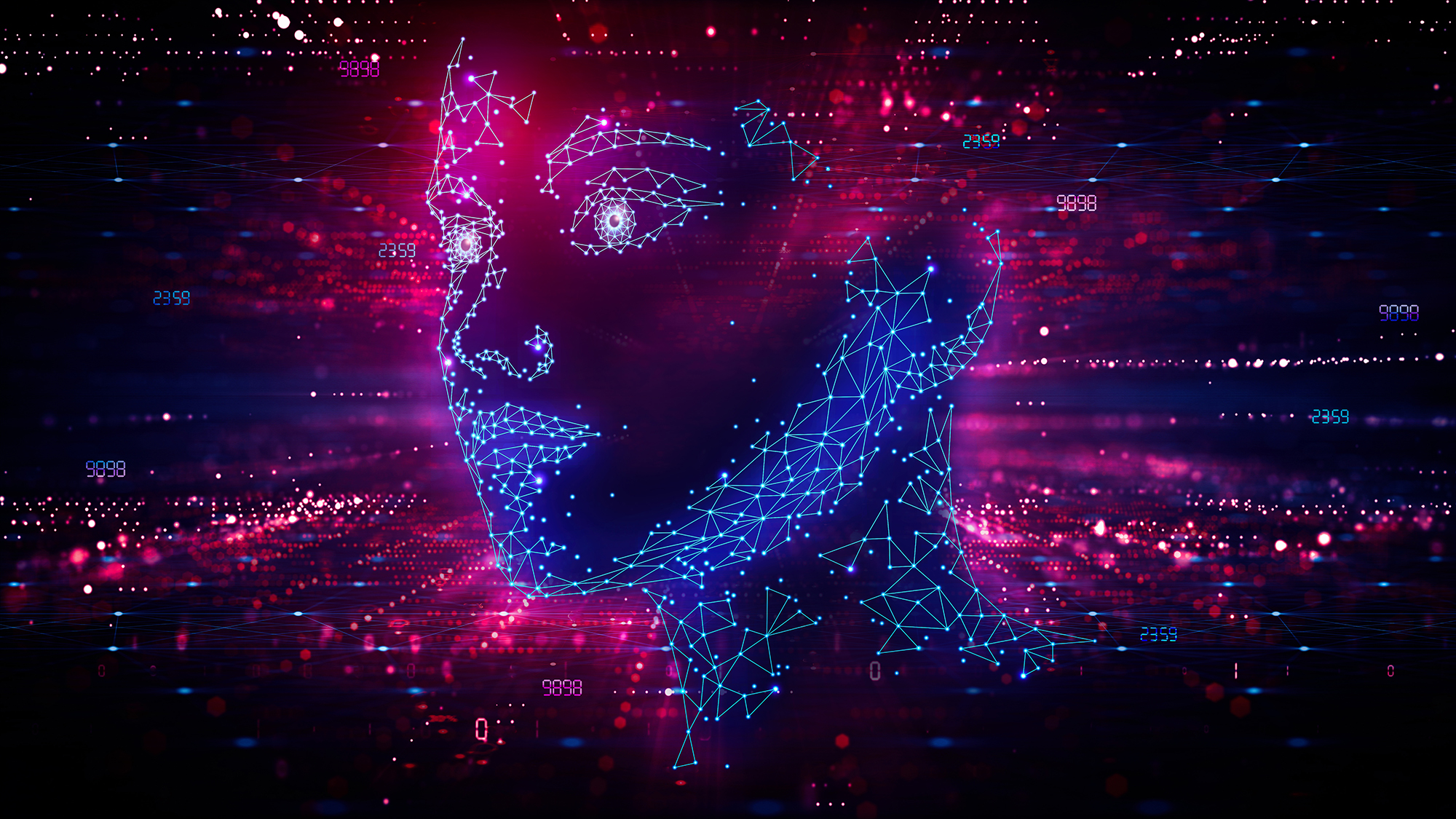
The cost to prompt high-end AI LLMs has plummeted from $20 per million tokens to $0.07 per million in just 18 months, according to Stanford's 2025 AI Index Report. A panoramic view of the worldwide AI landscape, Stanford's annual report also reports a serious need for more responsible AI guardrails and a tightening race between the US and China's emerging AI tech.
Stanford University's Institute for Human-Centered AI (HAI) has published its annual AI Index Report since 2017, with its recent reports regularly cited by world governments. HAI has collected and collated data on AI's myriad facets, studying investments into the market, where and how the tech is most used, and where it is most lacking. This year's report offers serious insights on the growth of AI over 2024, and predicts where it will likely go next.
AI Costs are lower... and higher
Artificial intelligence models have become significantly cheaper to use in only the last year — but at the same time they have become more expensive to train. This apparent contradiction is illustrated in HAI's helpful graphs accompanying the study: as major companies have ballooned their investments into their flagship models, the cost to operate and query the same models has dropped significantly.
The cost to query an AI is down, though, mostly because of better hardware, performance, and energy efficiency. (4/13) pic.twitter.com/7xXj680LegApril 8, 2025
OpenAI, Meta, and Google have all measurably increased the costs invested into their flagship language models. On average, each company spent 28 times as much money training its most recent flagship AI model as it did training the predecessor (Meta's $3 million to $170 million jump was the largest). Other relative newcomer, such as Mistral and xAI have also entered the game spending high — Grok-2 cost an estimated $107 million to train.
The cost to train these LLMs does not seem to be dropping anytime soon, either. xAI's Grok-3, which released to the public in February, is claimed to have used 10 times the GPUs of Grok-2's training. Grok-3 had no official price tag, but it could potentially have cost $1 billion or more to complete.
If these numbers to train a computer program seem astronomical, it's because they are. While these trillion-dollar companies invest hundreds of billions into the next generation of AI, the price to reach GPT-3.5 performance has shrunk. The cost to inference a model at GPT-3.5-level performance — defined by HAI as 64.8% accuracy — fell 280 times from November 2022 to October 2024.
Falling hardware and operation costs of smaller AI models contributed heavily to this price drop. Enterprise AI hardware costs have fallen 30% in the last year, with new hardware also being 40% more energy efficient. Companies are likely to continue spending more and more money on training flagship models every year, but typical users content with GPT-3.5 performance will find their costs becoming lower and lower.
China is catching up to U.S. dominance
The United States has been the highest spender and top performer in artificial intelligence since the tech's breakthrough into the mainstream. However, China is close behind on the AI race. The top performing U.S.- and China-based LLMs are getting closer and closer in performance when tested in industry benchmarks.
Previously, the U.S. had not only the most models, but also the best performing. This year, #China narrowed down that gap. In January 2024, the top U.S. model outperformed the best Chinese model by 9.26 percent; by February 2025, it was 1.70 percent. (6/13) pic.twitter.com/BY4ageBNrtApril 8, 2025
As the above graph displays, the U.S.'s best model only beat China's champion by 1.70%, as voted on by blind trials in LMSYS Chatbot Arena. Results from top benchmarks MMLU and HumanEval have also begun to approach even results, with the U.S. still managing to stay barely ahead.
The United States still handily beat China in quantity, if not quality. In a HAI collection of highly notably AI models, the United States took an easy lead with 40 of 2024's most notable LLMs. China fell distantly behind with 15, and all of Europe only contributed 3 models to the race.
Harmful AI incidents
HAI's chapter on Responsible AI paints a starker picture on the reality of using AI, which carries a non-zero level of risk. The AI Incident Database (AIID), a non-profit research organization dedicated to collecting information on harmful AI incidents, reportedly saw a disturbing increase in harmful AI incidents over 2024. 233 harmful or dangerous incidents were reported to the AIID in 2024, surpassing the ~150 reports in 2023 and ~100 in 2022.
Some of the most severe incidents in 2024 were listed in HAI's complete Chapter 3. These incidents included a false ID of a shopper thought to be a shoplifter with anti-theft AI, deepfake pornography, and instances of chatbots encouraging harmful behavior, including self-harm. Notably, few AI companies accept responsibility for AI incidents when they occur, with several of the above incidents leading to refusals to issue an apology or reparation from the involved companies.
Other insights
HAI's full 2025 AI Index can be found on the Stanford HAI website. The 8-chapter study covers a much broader range than could be covered here, representing many hours of reading. The AI landscape is broader and more invested in than ever before, making recent tariffs that threaten to shake up the status quo frightening to the still-nascent industry. The future of the tech is yet unknown, though hopefully safety and responsibility in training and application take up a more dominant share of attention in the coming years.