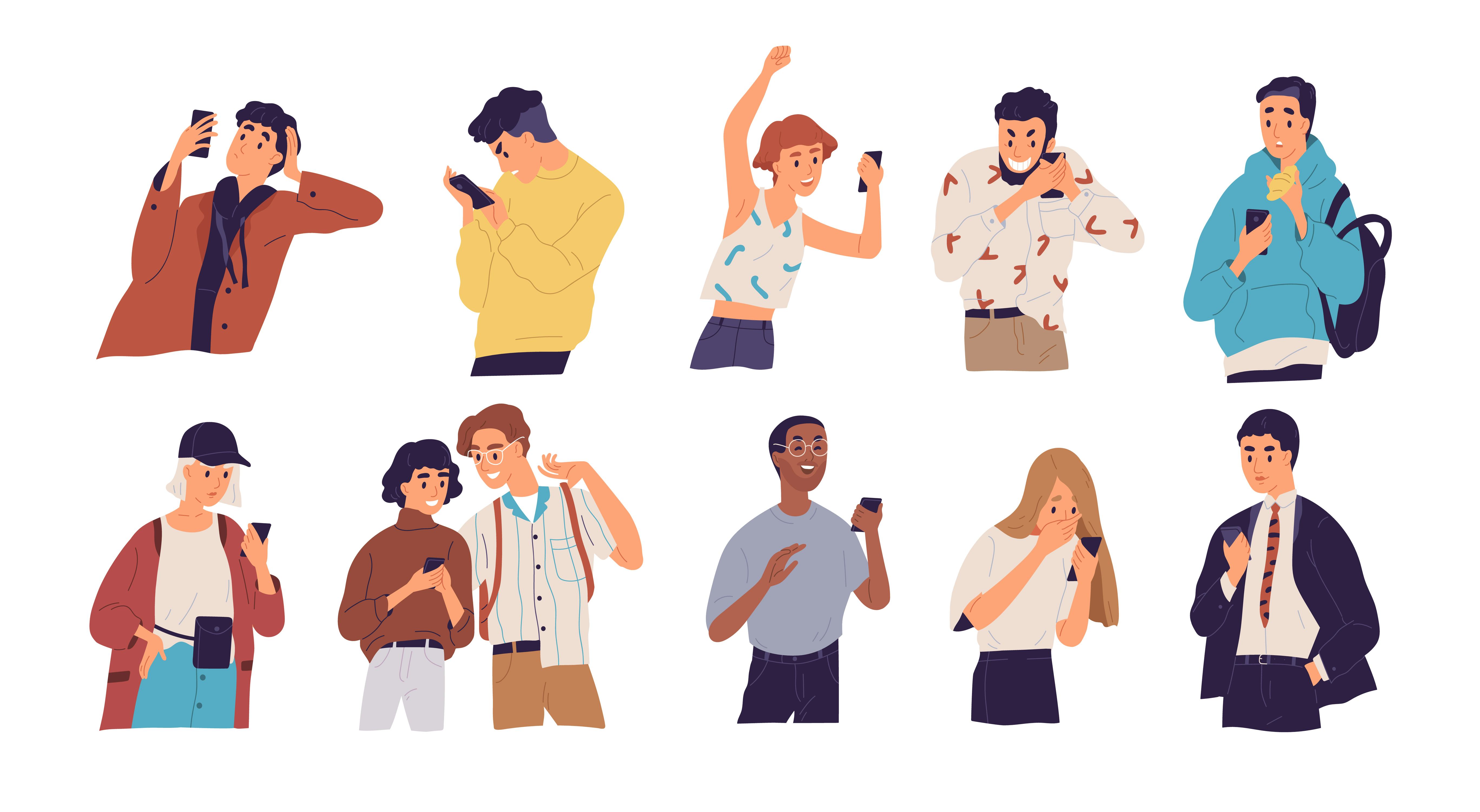
If someone took a magnifying glass to your Twitter feed, what kind of person would they find? Our social media feeds are a time-capsule of life's best (and worst) moments, including oversharing. New research from Stanford University and the National University of Singapore says that this data can be used to accurately predict our overall well-being.
Using machine learning and over 1.5 billion sample tweets, the research team looked at what word choice and usage said about well-being across the U.S. to see how these measurements of well-being compared to self-reported survey data. The researchers found that a data-driven analysis was able to more accurately predict people's well-being, but that wasn't all. They found that they could also determine biographical information like socioeconomic status or education.
The authors say that this trove of data could help them learn more about people's health conditions like sleep disorders and heart disease, as well as help communities recover from the emotional strain of Covid-19.
In their study, published Monday in the journal Proceedings of the National Academy of Sciences, researchers trained a machine learning model using over 2,000 Facebook posts to recognize the full vocabulary used on social media, including "netspeak" like 'lol' or 'blessed'. The authors write that this approach differs from word-level analysis which instead focuses on a "dictionary" (or collection of words) that are associated with specific emotional scores. To test the effectiveness of both methods the researchers compared their results to a national survey of well-being. Jaidka tells Inverse that this survey showed that people living on either the east or west coast tended to be overall happier and better off than those living in the mid-west.
"Words like ”lol” confound word-level methods"
Part of the challenge when it comes to analyzing modern language usage is that the same language can change its meaning between different regions or groups, says lead author and Assistant Professor of Computational Communication at the National University of Singapore, Kokil Jaidka, in a statement.
"How is internet use evolving the connotations of typically positive or negative words? And, how do these connotations change with culture and region? These are questions that need to be addressed before standard measurements can work as expected to estimate populations, and not merely individuals."
The researchers found that using emotion-coded words alone to evaluate communities' well-being created a greater discrepancy between the analysis and the survey results and that using a machine learning model to instead evaluate all a post's vocabulary was much more reflective of the true survey results. They also found that more native internet language (like 'lol' or 'lmao') tended to confuse the word-level models because they failed to recognize the different ways such words might be used in conversation.
"Words like ”lol” confound word-level methods because their contemporary use on social media is out of sync with their emotion scores in typical dictionaries, which interpret it as an expression of happiness," said Jaidka in a statement.
Removing these high-frequency "netspeak" words allowed for a higher correlation to the actual survey data.
Interestingly, the researchers found that false identification of words was most prevalent in samples coming from the south and southeast, with the word-level model often identifying words like "bless" or "faithful" as positive when they were actually used in a negative context. The researchers hypothesize that higher levels of religious language in this part of the country may explain why these words are used differently.
"Previous research has considered the South to be more religious than the North," Jaidka tells Inverse. "Although we find higher use of positive emotion words, and especially words related to religion, in the South, that didn't necessarily mean that they were happier than folks in the Silicon Valley who tweet about hangovers -- even though word-level methods would have predicted it to be so."
Their model was also able to identify socioeconomic differences based on word usage, such as the use of "mortgage" correlating to homeownership.
Based on the success of their data-driven model, Jaidka says the team is encouraged to continue pursuing how a machine learning algorithm like this could better understand linguistic nuances between different regions and people. Jaidka believes that improving these models could help improve the well-being of these communities as well.
This is not the first study looking at social media and mental health. Researchers have seen the utility of a public-facing database of emotions and words for years—a study from 2018 established that "social media platforms could further our understanding of schizophrenia."
"In the Covid-19 era that we are living in today, social media posts can help us understand how people are adapting to and coping with the new normal," Jaidka tells Inverse. "Our words are useful not just to understand what we -- as individuals -- think and feel -- but also the communities we live in. Our study humbly contributes better methods to unobtrusively measure people's mental and emotional health through social media posts ... [to] help governments to plan for better support systems, better infrastructure, and better techniques for interventions and outreach."
Abstract: Researchers and policy makers worldwide are interested in measuring the subjective well-being of populations. When users post on social media, they leave behind digital traces that reflect their thoughts and feelings. Aggregation of such digital traces may make it possible to monitor well-being at large scale. However, social media-based methods need to be robust to regional effects if they are to produce reliable estimates. Using a sample of 1.53 billion geotagged English tweets, we provide a systematic evaluation of word-level and data-driven methods for text analysis for generating well-being estimates for 1,208 US counties. We compared Twitter-based county-level estimates with well-being measurements provided by the Gallup-Sharecare Well-Being Index survey through 1.73 million phone surveys. We find that word-level methods (e.g., Linguistic Inquiry and Word Count [LIWC] 2015 and Language Assessment by Mechanical Turk [LabMT]) yielded inconsistent county-level wellbeing measurements due to regional, cultural, and socioeconomic differences in language use. However, removing as few as three of the most frequent words led to notable improvements in well-being prediction. Data-driven methods provided robust estimates, approximating the Gallup data at up to r = 0.64. We show that the findings generalized to county socioeconomic and health outcomes and were robust when poststratifying the samples to be more representative of the general US population. Regional well-being estimation from social media data seems to be robust when supervised data-driven methods are used.